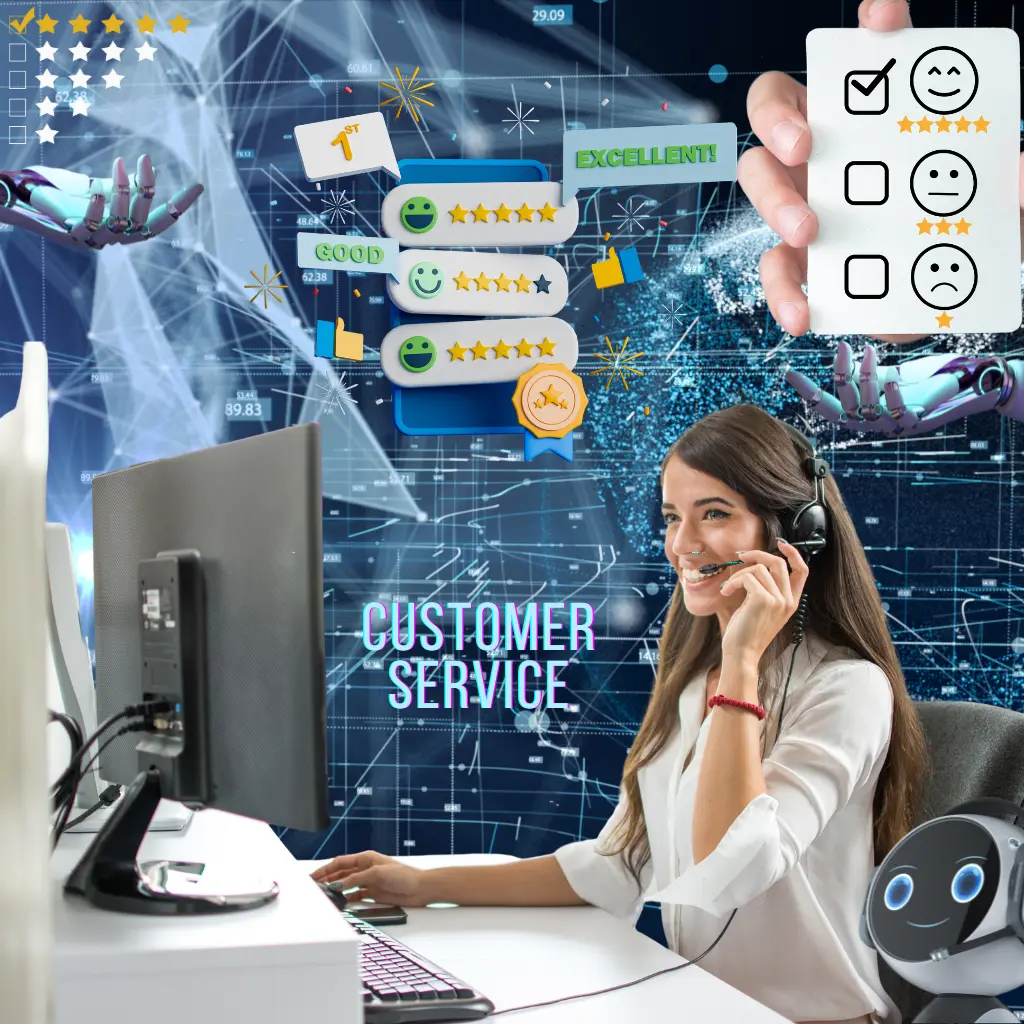
Revolutionizing Customer Service
Unleashing the Power of AI Tools in the Digital Domain
In the fast-evolving customer service landscape, integrating AI Tools for the Customer Services Domain has become a cornerstone for businesses aiming to enhance operational efficiency, elevate customer experiences, and stay at the forefront of technological innovation.
With a 15-year legacy in customer service, our company is poised to embark on a transformative journey by embracing a diverse array of AI technologies.
This discussion meticulously explores 50 distinct AI tools categorized into ten key domains, ranging from natural language processing (NLP) and speech recognition to predictive analytics, automation, facial recognition, and continuous learning.
Each tool is strategically designed to address specific facets of customer service, offering solutions that range from sentiment analysis and multilingual support to fraud detection and personalized training.
As we delve into the intricacies of these tools, we unravel a roadmap for revolutionizing customer service operations and solidifying our position as a market leader committed to innovation and excellence.
10 AI tool ideas for a SAAS platform catering to Customer Service startups, grouped by domain similarity:
1. Natural Language Processing (NLP) Tools:
- Text-based sentiment analysis tool: Utilizes NLP to analyze customer messages, emails, and reviews to determine the sentiment (positive, negative, or neutral).
- Chatbot for handling customer queries: Utilize NLP to understand and respond to the questions in natural language.
- Automated email response system: Uses NLP to understand and generate contextually appropriate responses to customer emails.
- Language translation tool for multilingual support: Utilizes machine translation powered by NLP to enable seamless communication in multiple languages.
- Automated content summarization tool: This tool applies NLP algorithms to condense and summarize large amounts of text content for efficient information retrieval.
2. Speech Recognition and Processing:
- Voice-based virtual assistant for phone support: It utilizes automatic speech recognition (ASR) to transcribe spoken words, enabling interaction through voice commands.
- Speech-to-text conversion for analyzing customer calls: Converts audio from customer calls into text for analysis using natural language understanding (NLU) techniques.
- Voice sentiment analysis for gauging customer emotions: Speech emotion recognition assesses the emotional tone in customer interactions.
- Automated call routing based on spoken keywords: Employs speech recognition to identify keywords and route calls to the appropriate departments.
- Speech analytics for quality monitoring: Analyzes recorded calls using AI to identify patterns, issues, and opportunities for improvement.
3. Predictive Analytics and Recommendation Systems:
- Customer behaviour prediction tool: This technology employs machine learning algorithms to forecast customer behaviour by analyzing past data and interactions.
- Product recommendation engine: This system leverages collaborative filtering and other recommendation algorithms to suggest products or services based on customer preferences.
- Forecasting tool for service demand: Uses predictive analytics to anticipate customer service demand and allocate resources accordingly.
- Predictive analytics for identifying potential issues: Machine learning is applied to identify and address potential problems before they impact customers.
- Churn prediction tool for proactive customer retention: This tool utilizes machine learning models to predict customers at risk of churning, enabling proactive retention efforts.
4. Automation and Robotic Process Automation (RPA):
- Automated ticket resolution system: This system employs RPA to automate the resolution of common customer issues by interacting with backend systems.
- RPA for repetitive task automation: It uses robotic process automation to streamline repetitive tasks, improve efficiency, and reduce manual efforts.
- Intelligent workflow automation: Utilizes AI to automate complex business processes and workflows intelligently.
- Automated follow-up on customer requests: Implements AI-driven automation to follow up on customer requests and ensure timely resolution.
- Process automation for order fulfilment: Applies RPA to automate order processing and fulfilment tasks in the customer service pipeline.
5. Knowledge Management Systems:
- AI-driven knowledge base for self-service: This utilizes AI algorithms to organize and update a knowledge base, enabling customers to find information independently.
- Content categorization and tagging tool: This tool applies machine learning to categorize and tag content, automatically improving knowledge base organization.
- Knowledge extraction from unstructured data: This approach employs NLP and ML algorithms to extract meaningful insights.
- Automated content updates based on user feedback: This system incorporates AI to analyze user feedback and automatically update and improve knowledge base content.
- Intelligent search and retrieval system: This system implements AI-enhanced search algorithms to retrieve information from the knowledge base accurately and efficiently.
6. Customer Feedback and Survey Analysis:
- Sentiment analysis of customer feedback: Employs NLP and machine learning to analyze and categorize sentiments expressed in customer feedback.
- Automated survey response analysis: Uses AI to analyze and interpret responses from customer surveys automatically.
- NLP-based feedback summarization: Utilizes natural language processing to summarize and extract critical insights from lengthy customer feedback.
- Customer satisfaction prediction tool: Applies machine learning to predict customer satisfaction levels based on feedback and interactions.
- Trend analysis in customer feedback: Utilizes AI to identify and analyze trends in customer feedback, helping in strategic decision-making.
7. Social Media Monitoring and Engagement:
- Social media sentiment analysis tool: Employs NLP and machine learning to analyze sentiment in social media mentions, comments, and messages.
- Automated response to social media queries: Uses chatbots and AI-driven systems to respond automatically to customer inquiries on social media platforms.
- Trend identification in social media conversations: Applies machine learning to identify and capitalize on emerging trends in social media discussions.
- Customer engagement analytics: Utilizes AI to analyze and optimize customer engagement strategies on social media.
- Automated posting and scheduling on social media platforms: This implementation uses AI algorithms to schedule and post content on social media platforms at optimal times.
8. Facial Recognition and Emotion Analysis:
- Facial recognition for identity verification: Utilizes facial recognition technology for secure customer identity verification.
- Emotion analysis in video customer interactions: Applies facial expression analysis to gauge customer emotions during video interactions.
- Biometric authentication for support access: Utilizes facial recognition and other biometric data for secure access to customer support services.
- Visual sentiment analysis in customer interactions: This technique employs computer vision techniques to analyze images or video content for sentiment and context.
- Security measures using facial recognition: Enhances security protocols by implementing facial recognition as an authentication measure.
9. Data Security and Privacy Tools:
- AI-based fraud detection system: Utilizes machine learning algorithms to detect patterns indicative of fraudulent activities in customer interactions.
- Privacy compliance monitoring tool: Applies AI to monitor and ensure compliance with data privacy regulations.
- Anomaly detection for unusual customer behaviour: Machine learning is used to identify anomalies in customer behaviour that may indicate security threats or unusual activities.
- Customer data encryption and protection: Implements AI-driven encryption and protection mechanisms to safeguard customer data.
- AI-driven security incident response system: This system uses AI to monitor and respond to security threats in real time, improving overall cybersecurity.
10. Continuous Learning and Improvement:
- AI-powered training and onboarding systems utilize machine learning to personalize training materials and adapt training programs based on individual performance.
- Feedback loop for model improvement: Establish a continuous feedback loop to collect user feedback and improve the performance of AI models.
- Continuous learning algorithms for evolving customer needs: Machine learning algorithms adapt to changing customer preferences and market trends.
- Automated performance analysis and improvement suggestions: AI is employed to analyze performance metrics and provide automated recommendations for improvement.
- AI-driven A/B testing for customer service strategies: Implements A/B testing using AI algorithms to experiment and optimize customer service strategies in real time.
Speech Recognition and Processing
Business Knowledge Required:
- Customer Service Operations: In-depth understanding of customer service workflows, including common issues, processes, and pain points.
- Call Center Dynamics: Knowledge of call centre operations, call handling protocols, and customer interaction scenarios.
- Emotional Intelligence in Customer Service: Understanding of emotional cues in customer interactions and how they impact customer satisfaction.
- Keyword Identification in Customer Queries: Knowledge of business-specific keywords and phrases relevant to customer inquiries and issues.
Software Knowledge Needed:
- Automatic Speech Recognition (ASR): Expertise in ASR technologies to accurately transcribe spoken words into text.
- Natural Language Understanding (NLU): Proficiency in NLU techniques to analyze transcribed text and extract meaning from customer calls.
- Speech Emotion Recognition: Knowledge of speech emotion recognition algorithms to assess the emotional tone in customer interactions.
- Speech Analytics Algorithms: Understanding speech analytics algorithms for identifying patterns, issues, and opportunities in recorded calls.
- Automated Call Routing Systems: We have expertise in developing speech recognition systems that identify keywords and route calls to appropriate departments.
Hardware Needed to Run the Tools:
- High-Performance Servers: These are potent servers with GPUs that can handle the computational demands of speech recognition, emotion analysis, and analytics.
- Storage Systems: Sufficient storage capacity to store and manage large volumes of recorded customer calls for analysis.
- Cloud Computing Resources: Leveraging cloud services for scalability and flexibility in processing customer calls.
Training Required to Run These Tools Once Created:
- User Interface Training: This training allows customer service representatives to interact with the voice-based virtual assistant and utilize automated call routing features.
- System Maintenance Training: IT personnel will be trained on system maintenance, troubleshooting, and updates for the AI Tools for the Customer Services Domain.
Various Integrations Necessary for These Tools to Execute:
- Call Center Software Integration: Integration with existing call centre software and CRM systems for seamless customer data access.
- Speech Analytics Platforms: Integration with speech analytics platforms enhances quality monitoring capabilities.
- Automated Call Routing Integration: Integration with call routing systems to ensure effective and accurate routing based on identified keywords.
Comparative Tools Already Available in the Market:
- Voice-based Virtual Assistant:
- Speech-to-Text Conversion:
- Speech Emotion Recognition:
- Automated Call Routing Systems:
- Speech Analytics Platforms:
Recommendation:
Considering the complexity and specialized nature of Speech Recognition and Processing tools, a combination of buying and customizing existing solutions is recommended:
Buy and Customize:
- Purchase existing solutions with proven performance in voice-based virtual assistants, speech-to-text conversion, emotion recognition, call routing, and speech analytics.
- Customize these tools to align with the specific needs and workflows of the Customer Services Domain.
Cost/Benefits Analysis on the Recommendation:
- Benefits:
- Time Efficiency: Buying existing tools reduces development time compared to building from scratch.
- Proven Performance: Commercial tools come with proven track records, ensuring reliability.
- Customization Control: Customization allows tailoring the tools to fit unique business requirements.
- Costs:
- Licensing Fees: Ongoing licensing fees for using commercial tools.
- Customization Expenses: Costs associated with tailoring the purchased tools to align with specific business needs.
Overall: The buy-and-customize approach balances between leveraging proven technologies and tailoring them to the unique requirements of AI Tools for the Customer Services Domain. This approach will likely result in a quicker and more cost-effective implementation while meeting the business’s specific needs.
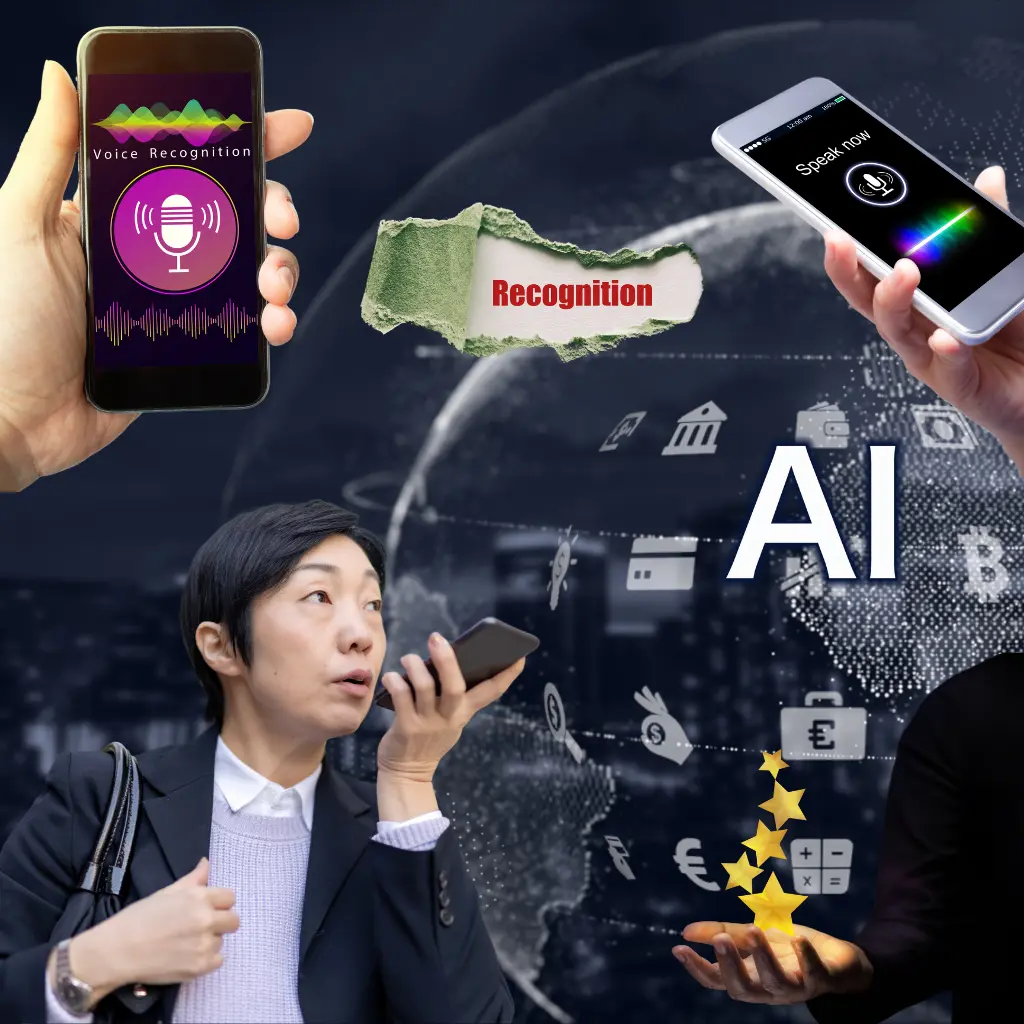
5 Customer Service Speech Recognition and Processing Tools
This discussion explores the integration of Customer Service Speech Recognition and Processing Tools in the customer service domain. Covering business, software, and hardware aspects, it aims to provide insights into the potential of these tools, compare market offerings, and offer recommendations for optimal implementation in our operations.
Natural Language Processing (NLP) Tools
Business Knowledge Required:
- Customer Service Domain Expertise: Understanding customer service workflows, joint issues, and the nuances of customer communication to tailor AI tools effectively.
- Sentiment Analysis Domain Knowledge: Grasping the intricacies of sentiment analysis, including language nuances, industry-specific sentiments, and context interpretation.
- NLP Algorithm Understanding: In-depth knowledge of Natural Language Processing algorithms to design and implement accurate sentiment analysis and chatbot functionalities.
- Multilingual Support Expertise: Understanding of linguistic challenges and variations to ensure effective language translation and support for diverse customer bases.
- Text Summarization Techniques: Understanding various NLP-based summarization techniques to create an automated content summarization tool that captures essential information.
Software Knowledge Needed:
- NLP Libraries/Frameworks: Proficiency in using NLP libraries such as NLTK, SpaCy, or TensorFlow for sentiment analysis, chatbot development, and email response generation.
- Machine Translation Tools: Knowledge of machine translation tools like OpenNMT or Google Translate API for creating language translation tools within the AI tools for the Customer Services Domain.
- Content Summarization Algorithms: Expertise in implementing extractive or abstractive summarization algorithms using tools like Gensim or BERT for the automated content summarization tool.
- API Integration Skills: The ability to integrate AI tools seamlessly with existing systems, CRM software, and communication platforms.
Hardware Needed to Run the Tools:
- High-Performance Servers: Powerful servers with GPUs to handle the computational demands of NLP and machine translation tasks for AI Tools for the Customer Services Domain.
- Cloud Computing Resources: Leveraging cloud services (e.g., AWS, Azure) for scalability, allowing the tools to handle varying workloads efficiently.
Training Required to Run These Tools Once Created:
- User Interface Training: Empower your customer service team to utilize AI tool user interfaces efficiently. They can confidently handle various customer inquiries and deliver exceptional service by providing the necessary training. This investment in their education will improve the customer experience and streamline your business operations.
- Maintenance Training: Training IT personnel to maintain and update the AI tools and address any issues or improvements.
Various Integrations Necessary for These Tools to Execute:
- CRM Integration: This feature will allow for a smooth integration with your customer relationship management (CRM) software. It will enable easy access to customer data and history, allowing for a seamless experience.
- Email and Communication Platform Integration: Integration with email systems and communication platforms to ensure synchronized responses and interactions.
- Language Database Integration: Integration with language databases for accurate translation and understanding.
Comparative Tools Already Available in the Market:
- Sentiment Analysis Tools:
- Chatbot Platforms:
- Email Response Automation:
- Language Translation Tools:
- Content Summarization Tools:
Recommendation:
Considering the specific requirements of AI Tools for the Customer Services Domain, a recommended approach would be a combination of buying and customizing:
Buy and Customize:
- Purchase existing tools with proven performance in sentiment analysis, chatbots, email response, language translation, and content summarization.
- Customize these tools to align with the company’s unique customer service processes and domain-specific requirements.
Cost/Benefits Analysis on the Recommendation:
- Benefits:
- Faster Deployment: Buying existing tools accelerates deployment compared to building from scratch.
- Reduced Development Costs: Purchasing established tools may be more cost-effective than developing complex NLP functionalities in-house.
- Proven Performance: Existing tools in the market often come with proven track records and reliability.
- Costs:
- Customization Expenses: Customizing the purchased tools may incur additional expenses for aligning them with the specific needs of the Customer Service Domain.
- Licensing Fees: Ongoing licensing fees for using commercial AI tools.
Overall: The buy-and-customize approach provides a balance between leveraging existing, proven solutions and tailoring them to the unique requirements of the Customer Service Domain. This strategy will likely result in a quicker and more cost-effective implementation of AI Tools for the Customer Services Domain.
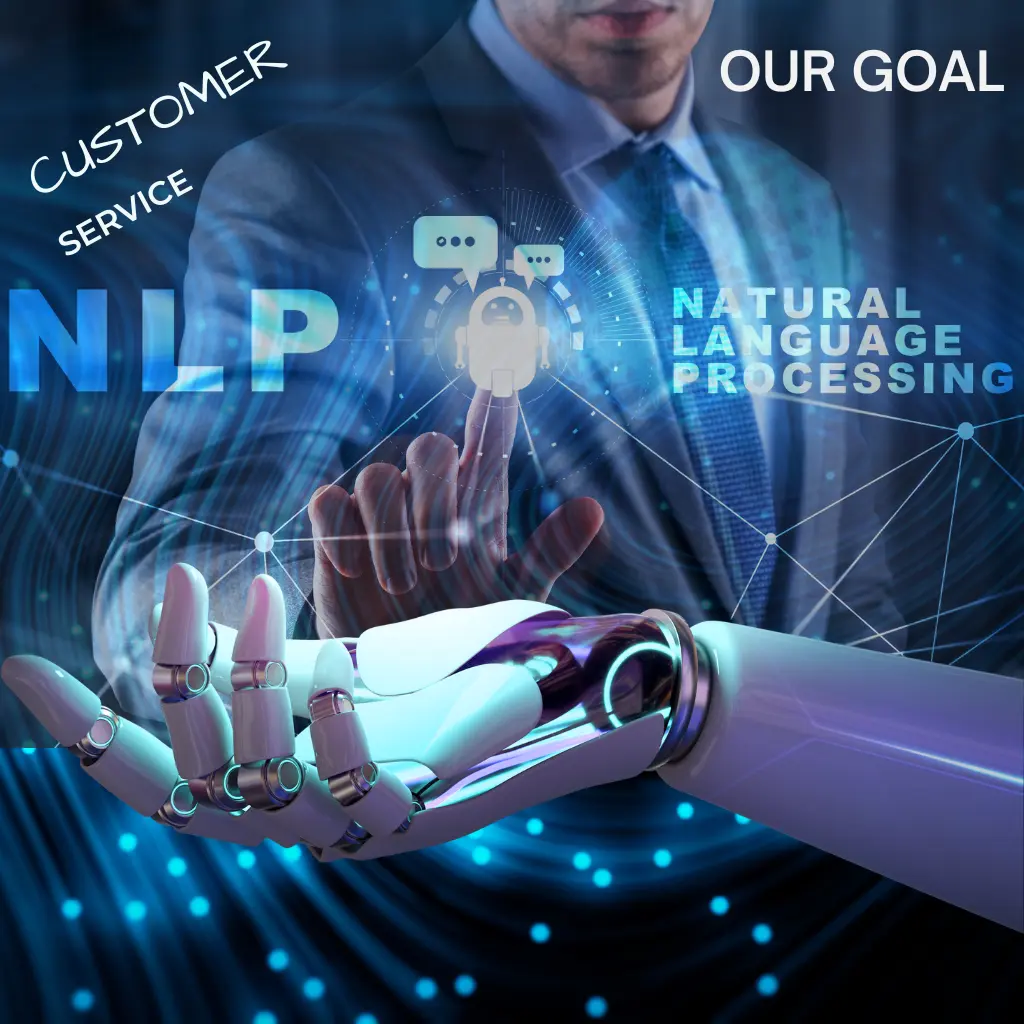
5 Customer Service Natural Language Processing (NLP) Tools
This discussion explores the fusion of business insight and software expertise required for successful implementation. These tools, spanning sentiment analysis, chatbots, email responses, language translation, and content summarization, aim to enhance customer interactions.
Predictive Analytics and Recommendation Systems
Business Knowledge Required:
- Customer Behavior Analysis: Deep understanding of customer behaviour patterns, preferences, and trends in the industry context.
- Product and Service Knowledge: In-depth knowledge of the company’s products and services to tailor recommendations based on customer preferences.
- Service Demand Dynamics: Insights into factors influencing customer service demand, historical service patterns, and seasonal variations.
- Customer Issue Identification: Knowledge of customers’ common issues, root causes, and patterns that may indicate potential problems.
- Customer Retention Strategies: Familiarity with customer retention strategies and the factors influencing customer churn in the industry.
Software Knowledge Needed:
- Machine Learning Algorithms: Expertise in selecting and implementing machine learning algorithms for behaviour prediction, collaborative filtering, forecasting, and issue identification.
- Collaborative Filtering Techniques: Understanding and implementing collaborative filtering algorithms for effective product and service recommendations.
- Predictive Analytics Tools: Proficiency in tools and platforms for predictive analytics to create the forecasting tool and identify potential issues.
- Churn Prediction Models: Knowledge of building and deploying machine learning models based on historical customer data to predict churn.
Hardware Needed to Run the Tools:
- High-Performance Servers: These servers have ample processing power to handle machine learning models.
- Data Storage Systems: Sufficient storage infrastructure to store and manage large volumes of historical customer data for analysis.
- Cloud Computing Resources: Cloud services should be utilized for scalability and flexibility, especially when dealing with variable workloads and data-intensive processes.
Training Required to Run These Tools Once Created:
- Data Science Training: This involves training data scientists and analysts on the tools and algorithms used for predictive analytics, behaviour prediction, and recommendation systems.
- User Interface Training: Customer service representatives will be trained on using the tools to enhance decision-making and customer interactions.
Various Integrations Necessary for These Tools to Execute:
- Customer Relationship Management (CRM) Integration: Integration with CRM systems to access and analyze customer data for behaviour prediction, recommendations, and issue identification.
- Inventory and Resource Management Integration: Integration with inventory and resource management systems to optimize resource allocation based on service demand forecasts.
- Marketing and Sales Integration: Integration with marketing and sales platforms to align predictive analytics with promotional activities and customer engagement strategies.
Comparative Tools Already Available in the Market:
- Customer Behavior Prediction:
- Product Recommendation Engines:
- Forecasting Tools:
- Churn Prediction Models:
Recommendation:
Considering the complexity and specialized nature of predictive analytics and recommendation systems, a combination of buying and customizing existing solutions is recommended:
Buy and Customize:
- Purchase proven tools with predictive analytics, recommendation systems, and churn prediction capabilities.
- Customize these tools to align with the unique requirements and data specifics of AI Tools for the Customer Services Domain.
Cost/Benefits Analysis on the Recommendation:
- Benefits:
- Time Efficiency: Buying existing tools accelerates deployment compared to building from scratch.
- Proven Performance: Commercial tools come with proven track records, ensuring reliability.
- Customization Control: Customization allows tailoring the tools to fit unique business requirements.
- Costs:
- Licensing Fees: Ongoing licensing fees for using commercial tools.
- Customization Expenses: Costs associated with tailoring the purchased tools to align with specific business needs.
Overall: The buy-and-customize approach balances between leveraging proven technologies and tailoring them to the unique requirements of AI Tools for the Customer Services Domain. This approach will likely result in a quicker and more cost-effective implementation while meeting the business’s specific needs.
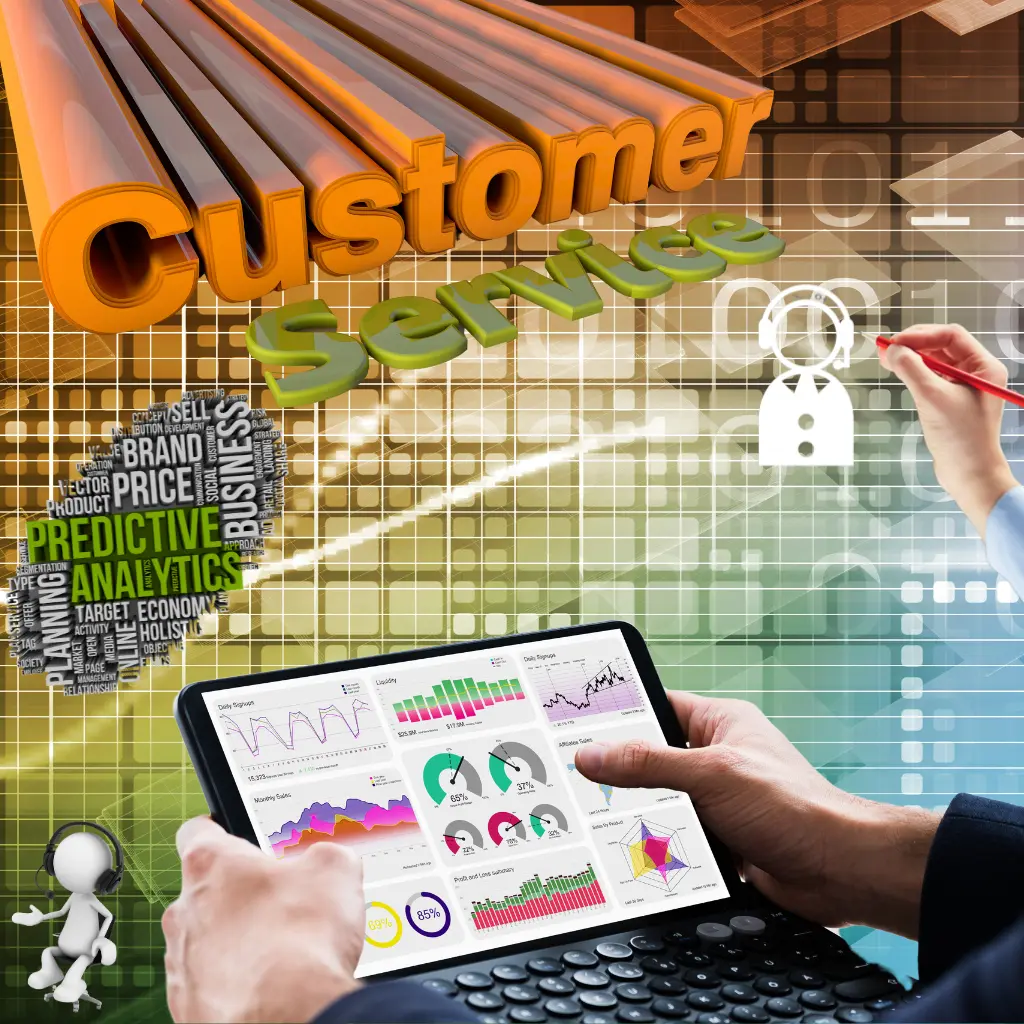
5 Customer Service Predictive Analytics and Recommendation
This transformative discussion aims to automate processes using advanced AI technologies to anticipate customer behaviours, proactively address issues, and optimize resource allocation for superior service excellence and customer satisfaction.
Automation and Robotic Process Automation (RPA)
Business Knowledge Required:
- Customer Service Operations: Deep understanding of customer service workflows, joint issues, and pain points to identify areas suitable for automation.
- Backend Systems Integration: Knowledge of the backend systems used in customer service to ensure seamless integration with the automated ticket resolution system.
- Repetitive Task Identification: Insight into daily repetitive tasks within the customer service domain that can be automated to enhance efficiency.
- Order Fulfillment Processes: Understanding the end-to-end order fulfilment processes, including order processing, inventory management, and delivery logistics.
- AI-Enhanced Follow-up Strategies: Knowledge of effective follow-up strategies in customer service and how AI can be leveraged to automate and improve this process.
Software Knowledge Needed:
- Robotic Process Automation (RPA) Tools: Proficiency in RPA tools such as UiPath, Automation Anywhere, or Blue Prism for building the automated ticket resolution system and repetitive task automation.
- Workflow Automation Platforms: Understanding workflow automation platforms like Zapier, Microsoft Power Automate, or tools to implement intelligent workflow automation.
- AI Integration Skills: Knowledge of AI integration techniques to infuse intelligence into workflow automation and follow-up processes.
Hardware Needed to Run the Tools:
- Servers and Cloud Resources: Robust servers or cloud resources with sufficient computing power to execute automated processes.
- Storage Systems: Adequate storage infrastructure to handle data storage and retrieval in automated processes.
- Networking Infrastructure: Stable and secure networking infrastructure for seamless communication between systems and tools.
Training Required to Run These Tools Once Created:
- RPA Tool Training: Personnel will receive training on using RPA tools to manage and maintain the automated ticket resolution and repetitive task automation systems.
- Workflow Automation Training: Training staff to understand and operate the intelligent workflow automation system.
- AI-Enhanced Follow-up System Training: This training is for customer service representatives to utilize the AI-driven follow-up system and interpret its outputs.
Various Integrations Necessary for These Tools to Execute:
- Backend System Integrations: Integration with backend systems (CRM, databases) for the automated ticket resolution system and order fulfilment automation.
- Communication Platform Integration: Integration with communication platforms for streamlined communication and follow-up on customer requests.
- Inventory Management System Integration: Integration with inventory management systems for order fulfilment automation.
Comparative Tools Already Available in the Market:
- RPA Tools:
- Workflow Automation Platforms:
- Order Fulfillment Automation Tools:
Recommendation:
Considering the maturity and availability of robust tools in the market, a recommendation would be to:
Buy and Customize:
- Invest in proven RPA tools and workflow automation platforms available in the market.
- Customize these tools to align with the specific needs and workflows of AI Tools for the Customer Services Domain.
Cost/Benefits Analysis on the Recommendation:
- Benefits:
- Time Efficiency: Buying existing tools accelerates deployment compared to building from scratch.
- Proven Performance: Commercial tools come with proven track records, ensuring reliability.
- Customization Control: Customization allows tailoring the tools to fit unique business requirements.
- Costs:
- Licensing Fees: Ongoing licensing fees for using commercial tools.
- Customization Expenses: Costs associated with tailoring the purchased tools to align with specific business needs.
Overall: The buy-and-customize approach balances between leveraging proven technologies and tailoring them to the unique requirements of AI Tools for the Customer Services Domain. This approach will likely result in a quicker and more cost-effective implementation while meeting the business’s specific needs.
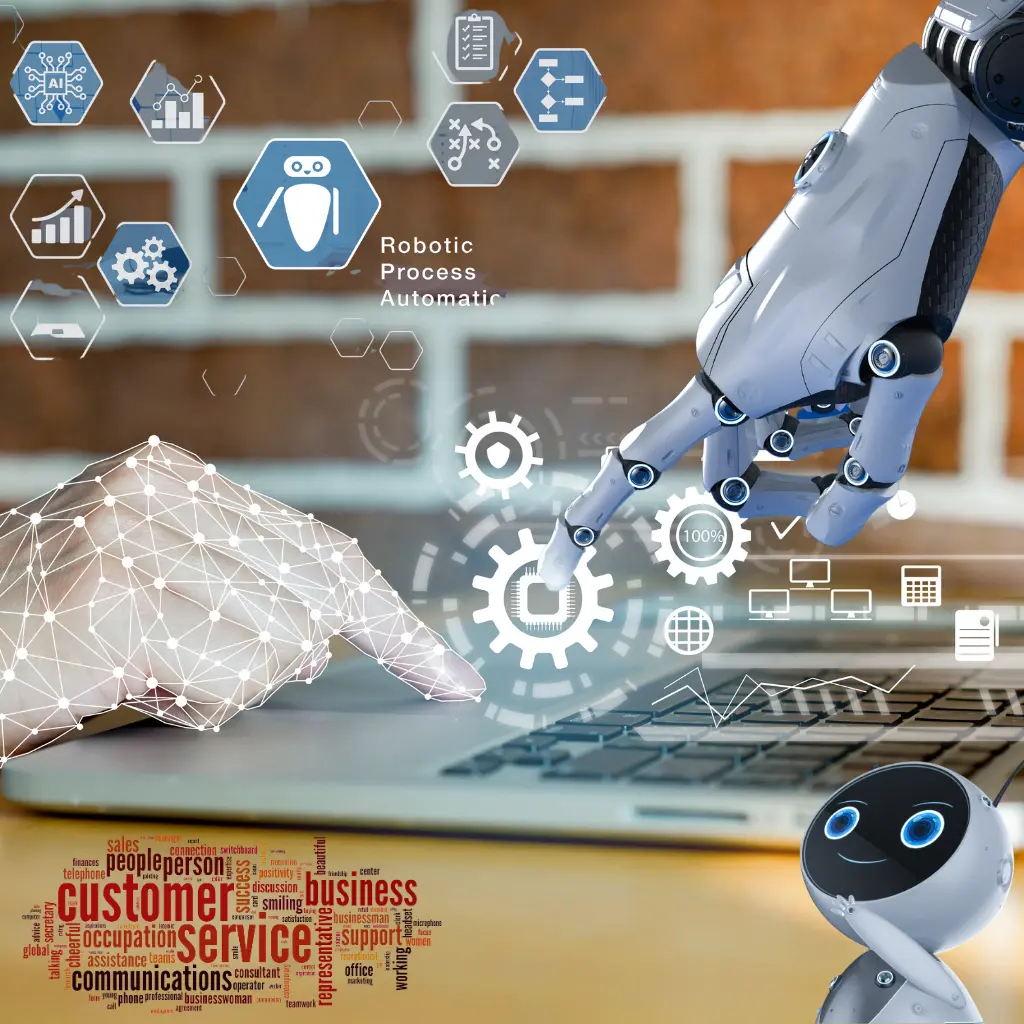
5 Customer Service Tolls for Automation and RPA
Embarking on a transformative journey to integrate 5 cutting-edge Customer Service Automation and Robotic Process Automation (RPA) Tools in the customer service domain. This discussion explores the requisite knowledge, hardware, training, and integrations, culminating in a strategic recommendation for optimal efficiency and customer-centric innovation.
Knowledge Management Systems
Business Knowledge Required:
- Knowledge Management in Customer Service: In-depth understanding of the customer service domain, including common queries, issues, and the type of information customers seek.
- User Behavior Analysis: Knowledge of user behaviour and preferences enhances the effectiveness of the knowledge base for self-service.
- Content Taxonomy and Categorization: Expertise in structuring content taxonomies and categories to ensure a logical organization within the knowledge base.
- Unstructured Data Understanding: Understanding unstructured data sources and the ability to identify relevant information from diverse content formats.
- User Feedback Analysis: Insight into customer feedback mechanisms and the ability to analyze feedback for knowledge base improvements.
Software Knowledge Needed:
- AI Algorithms for Knowledge Management: Proficiency in AI algorithms for knowledge base organization, user behaviour analysis, and automated content updates.
- Machine Learning for Content Categorization: Knowledge of machine learning techniques for automatically categorizing and tagging content within the knowledge base.
- Natural Language Processing (NLP): Expertise in NLP for knowledge extraction from unstructured data sources and improving search capabilities.
- AI-Driven Search Algorithms: Understanding of AI-enhanced search algorithms for efficient information retrieval from the knowledge base.
Hardware Needed to Run the Tools:
- Servers and Cloud Resources: Reliable servers or cloud resources with adequate computing power to handle the processing demands of AI algorithms and knowledge base storage.
- Storage Systems: Sufficient storage infrastructure to store the knowledge base content and user feedback data.
- Network Infrastructure: A robust network infrastructure to support seamless access and retrieval of information from the knowledge base.
Training Required to Run These Tools Once Created:
- Knowledge Management Training: Training for knowledge base administrators on updating and maintaining the knowledge base using AI-driven tools.
- User Training for Self-Service: Training for customer service representatives and end-users on utilizing the knowledge base for self-service.
Various Integrations Necessary for These Tools to Execute:
- Customer Relationship Management (CRM) Integration integrates CRM systems to incorporate customer data and history into the knowledge base.
- User Feedback Integration: Integration with feedback systems to capture and analyze user feedback for knowledge base improvements.
Comparative Tools Already Available in the Market:
- Knowledge Base Platforms:
- Content Categorization Tools:
- NLP and Knowledge Extraction Tools:
- AI-Driven Search Platforms:
Recommendation:
Considering the complexity and availability of robust tools in the market, a recommendation would be to:
Buy and Customize:
- Invest in proven knowledge base platforms and AI tools for content categorization, knowledge extraction, and search.
- Customize these tools to align with the specific needs and workflows of AI Tools for the Customer Services Domain.
Cost/Benefits Analysis on the Recommendation:
- Benefits:
- Time Efficiency: Buying existing tools accelerates deployment compared to building from scratch.
- Proven Performance: Commercial tools come with proven track records, ensuring reliability.
- Customization Control: Customization allows tailoring the tools to fit unique business requirements.
- Costs:
- Licensing Fees: Ongoing licensing fees for using commercial tools.
- Customization Expenses: Costs associated with tailoring the purchased tools to align with specific business needs.
Overall: The buy-and-customize approach balances between leveraging proven technologies and tailoring them to the unique requirements of AI Tools for the Customer Services Domain. This approach will likely result in a quicker and more cost-effective implementation while meeting the business’s specific needs.
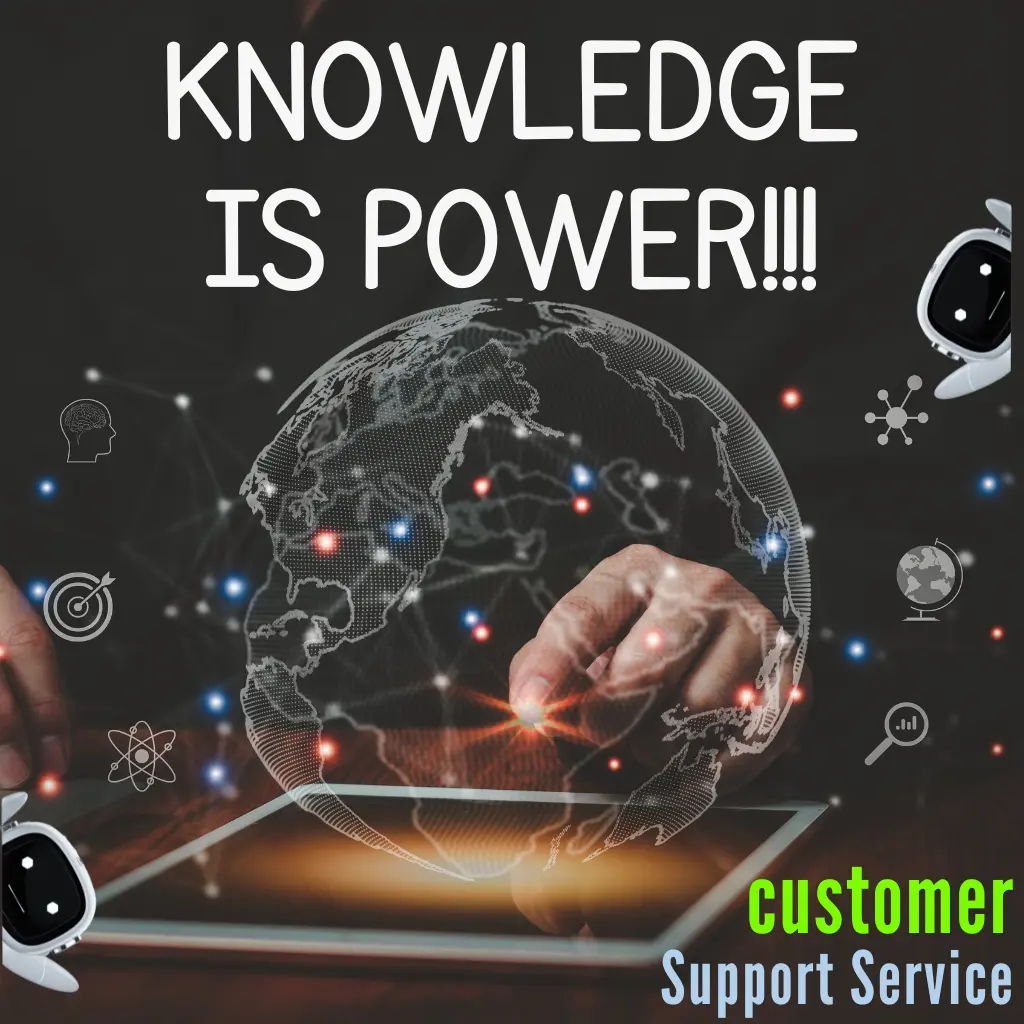
5 Customer Service Tools for Knowledge Management
This discussion focuses on the strategic adoption of Customer Service Tools for Knowledge Management, encompassing content categorization, knowledge extraction, automated updates, and intelligent search. The requisite business and software knowledge, hardware needs, training requirements, integrations, market tools, and a pragmatic recommendation for optimal efficiency and customer service excellence are explored.
Customer Feedback and Survey Analysis
Business Knowledge Required:
- Customer Service Processes: To align the tools with the business context, a deep understanding of customer service workflows, processes, and touchpoints is required.
- Customer Satisfaction Metrics: Knowledge of the key metrics used to measure customer satisfaction and the factors influencing it in the industry.
- Survey Design and Analysis: I have expertise in designing effective customer surveys, understanding survey methodologies, and interpreting survey results.
- Strategic Decision-Making: Awareness of how customer feedback contributes to strategic decision-making in the organization.
Software Knowledge Needed:
- Natural Language Processing (NLP): Proficiency in NLP for sentiment analysis, feedback summarization, and understanding natural language survey responses.
- Machine Learning Algorithms: I have expertise in implementing machine learning algorithms for sentiment prediction, customer satisfaction prediction, and trend analysis.
- Survey Analysis Tools: Knowledge of tools for automating the analysis of survey responses and extracting meaningful insights from structured and unstructured data.
- Data Visualization Tools: Familiarity with tools for visualizing data trends and insights from customer feedback.
Hardware Needed to Run the Tools:
- High-Performance Servers: These robust servers have ample processing power to handle the computational demands of sentiment analysis, machine learning, and survey analysis.
- Cloud Computing Resources: Leveraging cloud services for scalability and flexibility, especially during peak survey periods.
Training Required to Run These Tools Once Created:
- NLP and ML Training: This course is for data scientists and analysts. It focuses on the NLP and machine learning models used in sentiment analysis, satisfaction prediction, and trend analysis.
- User Interface Training: Training for customer service representatives or survey administrators on using the tools for data interpretation and decision-making.
Various Integrations Necessary for These Tools to Execute:
- Survey Platforms Integration: Integration with survey platforms seamlessly collects and analyses survey responses in real-time.
- CRM Integration: Integrating customer feedback into their CRM profiles is crucial.
Comparative Tools Already Available in the Market:
- Sentiment Analysis Tools:
- Survey Analysis Platforms:
- Customer Satisfaction Prediction Models:
Recommendation:
Considering the specialized nature of customer feedback and survey analysis tools, a recommendation would be to:
Buy and Customize:
- Invest in proven tools with capabilities in sentiment analysis, survey response analysis, and customer satisfaction prediction.
- Customize these tools to align with the specific needs and workflows of AI Tools for the Customer Services Domain.
Cost/Benefits Analysis on the Recommendation:
- Benefits:
- Time Efficiency: Buying existing tools accelerates deployment compared to building from scratch.
- Proven Performance: Commercial tools come with proven track records, ensuring reliability.
- Customization Control: Customization allows tailoring the tools to fit unique business requirements.
- Costs:
- Licensing Fees: Ongoing licensing fees for using commercial tools.
- Customization Expenses: Costs associated with tailoring the purchased tools to align with specific business needs.
Overall: The buy-and-customize approach balances between leveraging proven technologies and tailoring them to the unique requirements of AI Tools for the Customer Services Domain. This approach will likely result in a quicker and more cost-effective implementation while meeting the business’s specific needs.
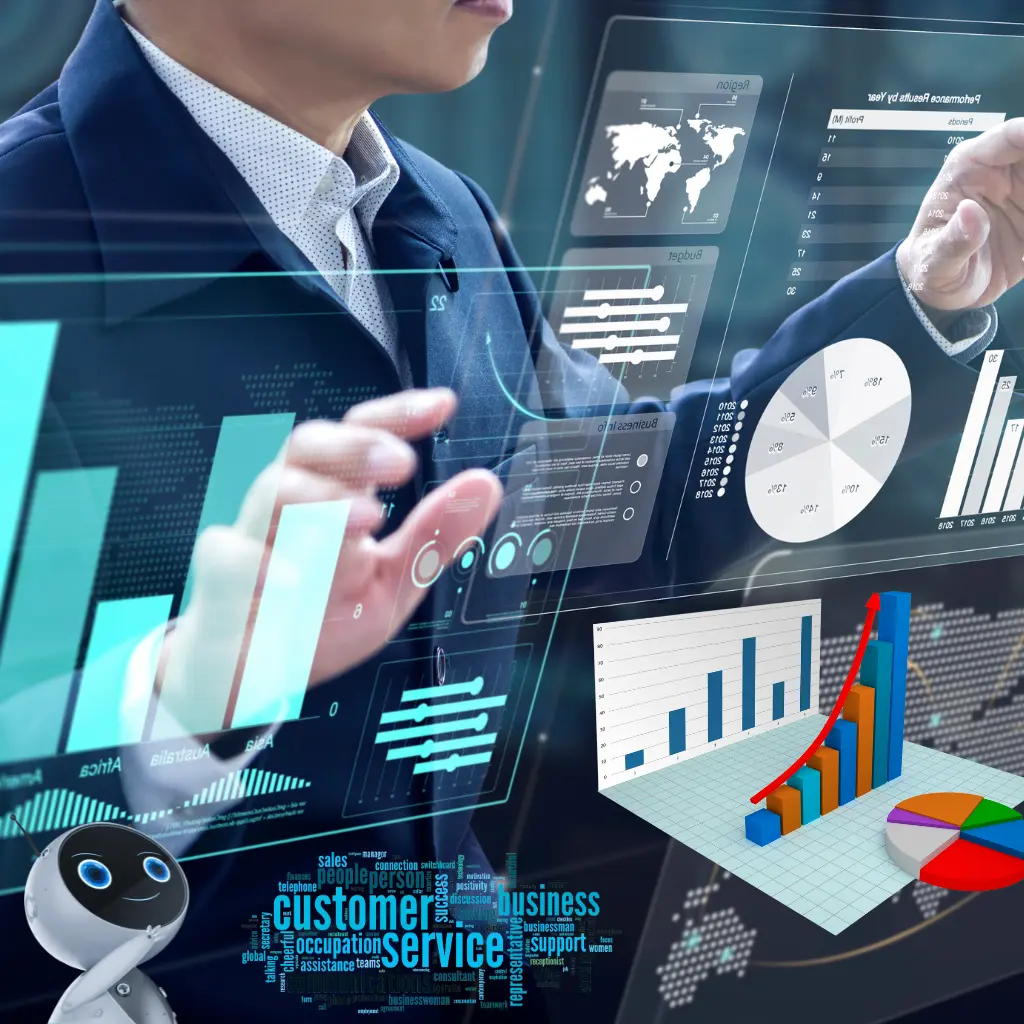
5 Customer Service Tools for Customer Survey Analysis
Our strategic initiative is implementing advanced customer service tools for feedback, survey analysis, and satisfaction prediction. This will revolutionize our customer service processes and enhance customer experience, leading to a better market position.
Social Media Monitoring and Engagement
Business Knowledge Required:
- Social Media Dynamics: In-depth understanding of how customer interactions and sentiments manifest on various social media platforms.
- Customer Engagement Strategies: Knowledge of effective customer engagement strategies and the factors contributing to positive social media interactions.
- Trend Identification: Awareness of industry trends and the ability to identify emerging trends from social media conversations.
- Chatbot Integration with Social Media: Understanding how chatbots can seamlessly integrate with different social media platforms for automated responses.
Software Knowledge Needed:
- NLP and Machine Learning for Sentiment Analysis: Proficiency in NLP and machine learning algorithms for sentiment analysis in social media mentions and comments.
- Chatbot Development: Expertise in chatbot development platforms and frameworks for creating AI-driven systems to respond to social media queries.
- Machine Learning for Trend Identification: Knowledge of machine learning techniques to identify and capitalize on emerging trends in social media discussions.
- Customer Engagement Analytics Tools: Familiarity with tools for analyzing customer engagement metrics on social media and optimizing strategies.
- Scheduling Algorithms for Social Media Posts: Understanding AI algorithms to determine optimal posting times and automate content scheduling on social media platforms.
Hardware Needed to Run the Tools:
- Servers and Cloud Resources: Robust servers or cloud resources with sufficient computing power to handle real-time analysis of social media data.
- Storage Systems: Adequate storage infrastructure to store and retrieve social media data for analysis.
- Networking Infrastructure: Stable and high-speed networking infrastructure to connect with various social media platforms.
Training Required to Run These Tools Once Created:
- Chatbot Training: Training for customer service representatives on handling customer interactions through the AI-driven chatbot system.
- Social Media Analyst Training: This training is for analysts using the tools to interpret sentiment analysis, trend identification, and engagement analytics.
Various Integrations Necessary for These Tools to Execute:
- Social Media Platform APIs: Integration with APIs of various social media platforms for data collection, posting, and automated responses.
- Customer Relationship Management (CRM) Integration: Integration with CRM systems to link social media interactions with individual customer profiles.
Comparative Tools Already Available in the Market:
- Social Media Sentiment Analysis:
- Chatbot Platforms:
- Trend Analysis Tools:
- Customer Engagement Analytics:
- Social Media Scheduling Tools:
Recommendation:
Considering the availability of specialized tools in the market, a recommendation would be to:
Buy and Customize:
- Invest in proven tools with capabilities in social media sentiment analysis, chatbot development, trend identification, customer engagement analytics, and social media scheduling.
- Customize these tools to align with the specific needs and workflows of AI Tools for the Customer Services Domain.
Cost/Benefits Analysis on the Recommendation:
- Benefits:
- Time Efficiency: Buying existing tools accelerates deployment compared to building from scratch.
- Proven Performance: Commercial tools come with proven track records, ensuring reliability.
- Customization Control: Customization allows tailoring the tools to fit unique business requirements.
- Costs:
- Licensing Fees: Ongoing licensing fees for using commercial tools.
- Customization Expenses: Costs associated with tailoring the purchased tools to align with specific business needs.
Overall: The buy-and-customize approach balances between leveraging proven technologies and tailoring them to the unique requirements of AI Tools for the Customer Services Domain. This approach will likely result in a quicker and more cost-effective implementation while meeting the business’s specific needs.
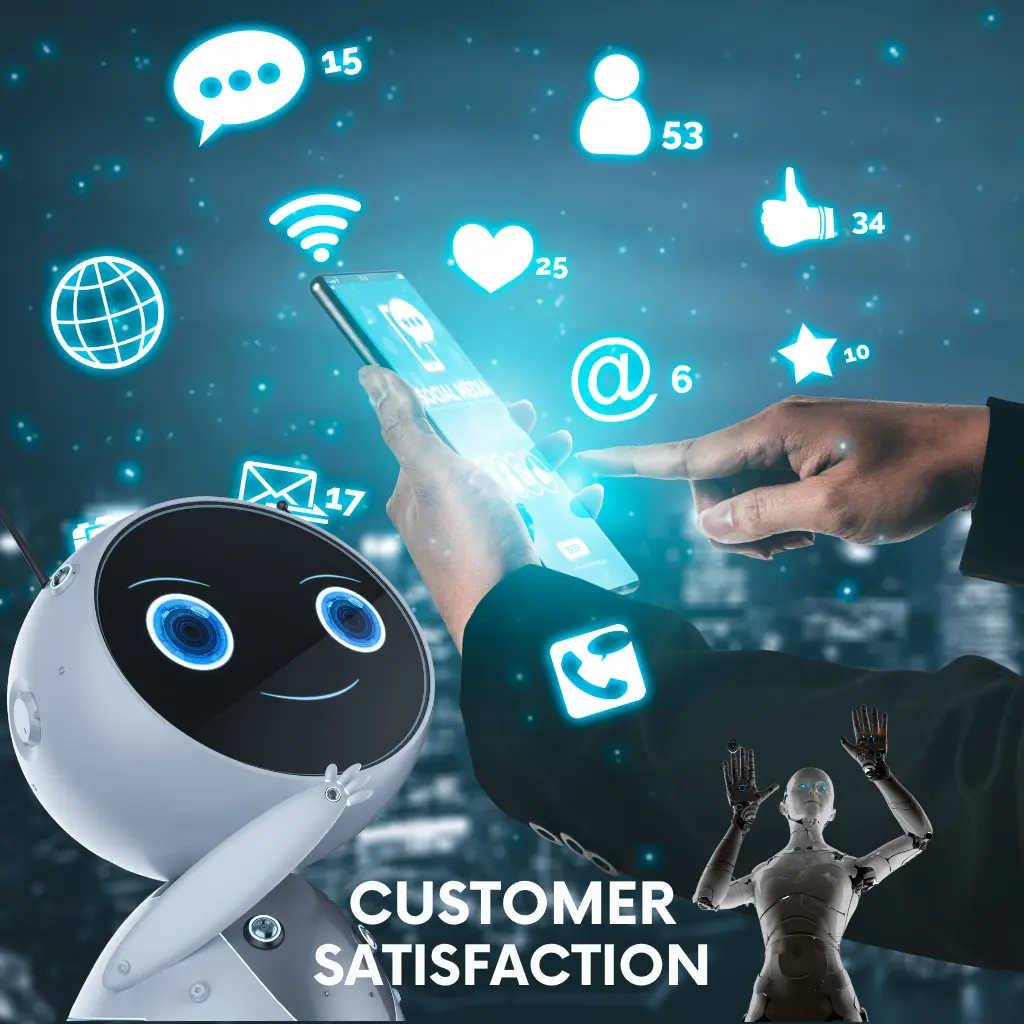
5 Customer Service Tools for Social Media Monitoring
This discussion explores the implementation of “Customer Service Tools for Social Media Monitoring and Engagement,” emphasizing the integration of AI technologies to optimize customer interactions. The platform offers efficient sentiment analysis, automated responses, trend identification, analytics, and posting for proactive engagement and elevated customer experiences.
Facial Recognition and Emotion Analysis
Business Knowledge Required:
- Identity Verification Processes: In-depth knowledge of existing identity verification processes and the regulatory requirements for customer authentication.
- Customer Support Security Protocols: Understanding of security measures in customer support and the potential vulnerabilities facial recognition can address.
- Customer Interaction Context: Knowledge of customer interactions in various forms (video, images) and understanding how emotions and sentiments play a role.
- Biometric Authentication Standards: Familiarity with biometric authentication standards and compliance requirements for customer support access.
Software Knowledge Needed:
- Facial Recognition Algorithms: Proficiency in facial recognition algorithms for identity verification, support access, and security measures.
- Emotion Analysis Models: Expertise in machine learning models for analyzing facial expressions to determine customer emotions during video interactions.
- Computer Vision Techniques: Knowledge of computer vision techniques for visual sentiment analysis in images or video content.
- Biometric Authentication Software: Understanding software tools for implementing secure biometric authentication measures.
Hardware Needed to Run the Tools:
- High-Performance Servers: These robust servers have powerful GPUs to handle facial recognition, emotion analysis, and computational demands for computer vision.
- Facial Recognition Cameras: Specialized cameras can capture high-quality images for accurate facial recognition.
- Biometric Authentication Devices: Biometric scanners or devices for capturing and verifying biometric data for authentication.
Training Required to Run These Tools Once Created:
- Security Training: Train security personnel to utilize facial recognition and biometric authentication tools for identity verification and access control.
- Customer Support Training: Customer support representatives will be trained on facial recognition and emotion analysis tools during video interactions.
Various Integrations Necessary for These Tools to Execute:
- Customer Support Systems Integration: Integration with customer support systems for biometric authentication and access control.
- Security Systems Integration: Integration with existing security systems to enhance overall security measures.
Comparative Tools Already Available in the Market:
- Facial Recognition Tools:
- Emotion Analysis Platforms:
- Biometric Authentication Solutions:
Recommendation (Build from Scratch/Buy from Market/buy and customize):
Considering the specialized nature and potential legal and regulatory implications, a recommendation would be to:
Buy and Customize:
- Invest in proven tools with capabilities in facial recognition, emotion analysis, and biometric authentication available in the market.
- Customize these tools to align with the specific security and customer service workflows of AI Tools for the Customer Services Domain.
Cost/Benefits Analysis on the Recommendation:
- Benefits:
- Time Efficiency: Buying existing tools accelerates deployment compared to building from scratch.
- Proven Performance: Commercial tools come with proven track records, ensuring reliability.
- Customization Control: Customization allows tailoring the tools to fit unique business requirements.
- Costs:
- Licensing Fees: Ongoing licensing fees for using commercial tools.
- Customization Expenses: Costs associated with tailoring the purchased tools to align with specific business needs.
Overall: The buy-and-customize approach balances between leveraging proven technologies and tailoring them to the unique requirements of AI Tools for the Customer Services Domain. This approach will likely result in a quicker and more cost-effective implementation while meeting the business’s specific needs.
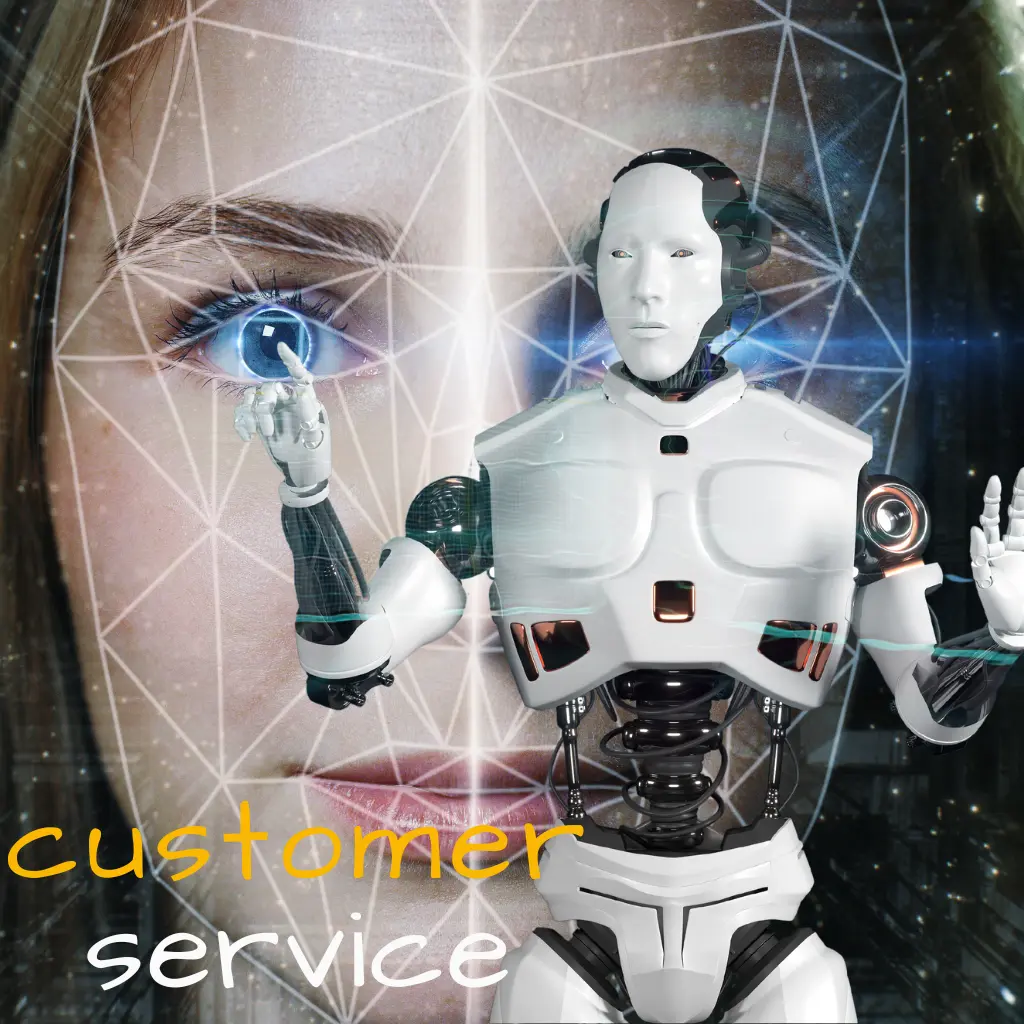
5 Facial Recognition and Emotion Analysis Tools of Customer Service
Embarking on a technological leap, our company pioneers Customer Service Tools for Facial Recognition and Emotion Analysis, poised to revolutionize customer interactions. This initiative integrates advanced technologies for identity verification, sentiment analysis, and enhanced security, ensuring a personalized, secure, and cutting-edge customer service experience.
Data Security and Privacy Tools
Business Knowledge Required:
- Fraud Detection in Customer Interactions: Understanding common fraud patterns and techniques used in customer interactions within the industry.
- Data Privacy Regulations: In-depth knowledge of data privacy regulations and compliance requirements applicable to customer service operations.
- Anomaly Detection for Security Threats: Awareness of potential security threats and anomalies in customer behaviour that may indicate security risks.
- Customer Data Protection Strategies: Expertise in customer data protection strategies and the legal and ethical considerations involved.
- Incident Response Protocols: Knowledge of incident response protocols and the specific requirements of the customer service domain.
Software Knowledge Needed:
- Machine Learning for Fraud Detection: Proficiency in machine learning algorithms for building a fraud detection system capable of identifying suspicious patterns.
- Privacy Compliance Monitoring Tools: Understanding AI tools for monitoring and ensuring compliance with data privacy regulations.
- Anomaly Detection Models: Expertise in building anomaly detection models to identify unusual customer behaviour indicating potential security threats.
- Encryption and Data Protection Mechanisms: Knowledge of AI-driven encryption techniques and data protection mechanisms for securing customer data.
- Security Incident Response Systems: Proficiency in building AI-driven systems for detection and response to security incidents.
Hardware Needed to Run the Tools:
- High-Performance Servers: These are robust servers with sufficient processing power to handle real-time fraud detection, anomaly detection, and incident response analysis.
- Data Encryption Hardware: Specialized hardware for implementing AI-driven encryption and protection mechanisms.
Training Required to Run These Tools Once Created:
- Fraud Detection Training: This training is for security personnel to interpret and respond to alerts generated by the fraud detection system.
- Privacy Compliance Training: Training personnel responsible for data privacy compliance to utilize the monitoring tool effectively.
- Anomaly Detection Training: Training for security analysts on interpreting results from the anomaly detection system and responding to potential threats.
Various Integrations Necessary for These Tools to Execute:
- Customer Relationship Management (CRM) Integration: Integration with CRM systems to link fraud detection and anomaly alerts with individual customer profiles.
- Data Privacy Compliance Integration: Integration with existing data privacy compliance systems and tools.
Comparative Tools Already Available in the Market:
- Fraud Detection Tools:
- Privacy Compliance Monitoring Tools:
- Anomaly Detection Platforms:
- Data Encryption and Protection Solutions:
- Security Incident Response Systems:
Recommendation:
Considering the critical nature of data security and privacy, a recommendation would be to:
Buy and Customize:
- Invest in proven tools available on the market that can detect fraud, monitor privacy compliance, detect anomalies, encrypt data, and respond to incidents.
- Customize these tools to align with the specific security and compliance needs of AI Tools for the Customer Services Domain.
Cost/Benefits Analysis on the Recommendation:
- Benefits:
- Time Efficiency: Buying existing tools accelerates deployment compared to building from scratch.
- Proven Performance: Commercial tools come with proven track records, ensuring reliability.
- Customization Control: Customization allows tailoring the tools to fit unique business requirements.
- Costs:
- Licensing Fees: Ongoing licensing fees for using commercial tools.
- Customization Expenses: Costs associated with tailoring the purchased tools to align with specific business needs.
Overall: The buy-and-customize approach balances between leveraging proven technologies and tailoring them to the unique requirements of AI Tools for the Customer Services Domain. This approach will likely result in a quicker and more cost-effective implementation while meeting the business’s specific needs.
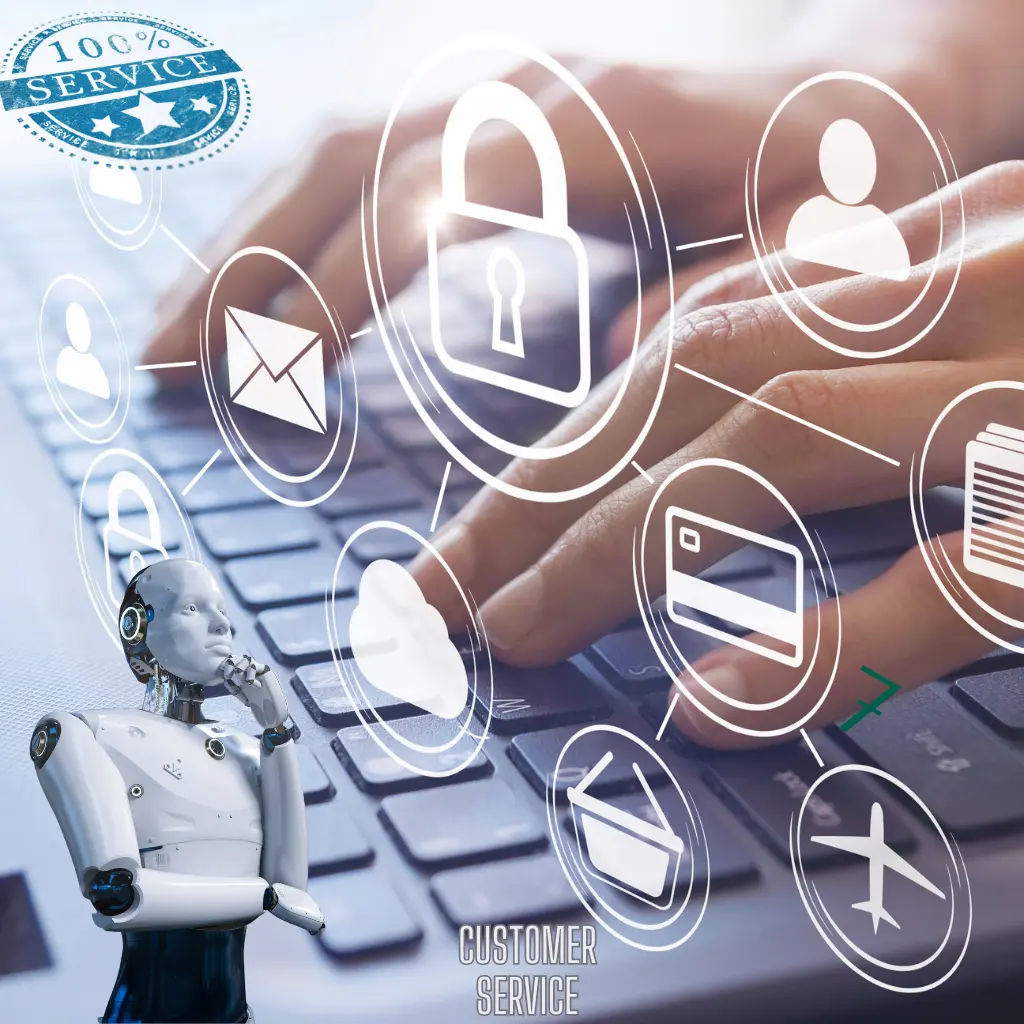
5 Customer Service Tools for Data Security and Privacy
This discussion delves into the imperative realm of Customer Service Tools for Data Security and Privacy, where advanced AI technologies are harnessed to automate processes. From fraud detection to anomaly identification, the focus is on building robust tools that fortify data security, ensuring customer trust in the ever-evolving landscape of customer service.
Continuous Learning and Improvement
Business Knowledge Required:
- Training and Onboarding Processes: In-depth understanding of existing training and onboarding processes within the customer service domain.
- User Feedback Mechanisms: Knowledge of effective mechanisms for collecting user feedback and insights from training employees.
- Customer Needs and Preferences: Insight into evolving customer needs, preferences, and market trends to adapt training and service strategies accordingly.
- Performance Analysis Metrics: Understanding key performance metrics relevant to customer service and how they impact overall performance.
- A/B Testing Strategies: Familiarity with A/B testing methodologies and strategies for optimizing customer service approaches.
Software Knowledge Needed:
- Machine Learning for Personalization: Proficiency in machine learning algorithms for personalizing training materials and adapting training programs based on individual performance.
- Feedback Loop Systems: Expertise in building feedback loop systems that collect, analyze, and incorporate user feedback to improve models.
- Continuous Learning Algorithms: Knowledge of constant learning algorithms that adapt to changing customer preferences and market trends.
- Performance Analysis Tools: Understanding AI-driven tools for analyzing performance metrics and providing automated improvement suggestions.
- A/B Testing Platforms: Proficiency in A/B testing platforms that use AI algorithms to experiment and optimize customer service strategies in real time.
Hardware Needed to Run the Tools:
- High-Performance Servers: These robust servers have ample processing power to handle machine learning algorithms, continuous learning, and A/B testing.
- Storage Systems: Adequate storage infrastructure for training materials, user feedback, and performance analysis data.
Training Required to Run These Tools Once Created:
- AI Training for Administrators: Provide administrators with training to oversee and manage AI-driven training and improvement tools.
- User Training for Feedback Participation: Training for employees and users on providing effective feedback to contribute to model improvement.
Various Integrations Necessary for These Tools to Execute:
- Learning Management System (LMS) Integration: Integration with existing LMSs to seamlessly incorporate AI-powered training.
- Performance Metrics Integration: Integration with performance metrics systems to collect and analyze relevant data for improvement suggestions.
Comparative Tools Already Available in the Market:
- Personalized Training Systems:
- Feedback Loop and Model Improvement Tools:
- Continuous Learning Platforms:
- Performance Analysis Tools:
- A/B Testing Platforms:
Recommendation:
Considering the complexity and availability of specialized tools in the market, a recommendation would be to:
Buy and Customize:
- Invest in proven tools with capabilities in personalized training, feedback loop and model improvement, continuous learning, performance analysis, and A/B testing available in the market.
- Customize these tools to align with the specific training and improvement needs of AI Tools for the Customer Services Domain.
Cost/Benefits Analysis on the Recommendation:
- Benefits:
- Time Efficiency: Buying existing tools accelerates deployment compared to building from scratch.
- Proven Performance: Commercial tools come with proven track records, ensuring reliability.
- Customization Control: Customization allows tailoring the tools to fit unique business requirements.
- Costs:
- Licensing Fees: Ongoing licensing fees for using commercial tools.
- Customization Expenses: Costs associated with tailoring the purchased tools to align with specific business needs.
Overall: The buy-and-customize approach balances between leveraging proven technologies and tailoring them to the unique requirements of AI Tools for the Customer Services Domain. This approach will likely result in a quicker and more cost-effective implementation while meeting the business’s specific needs.
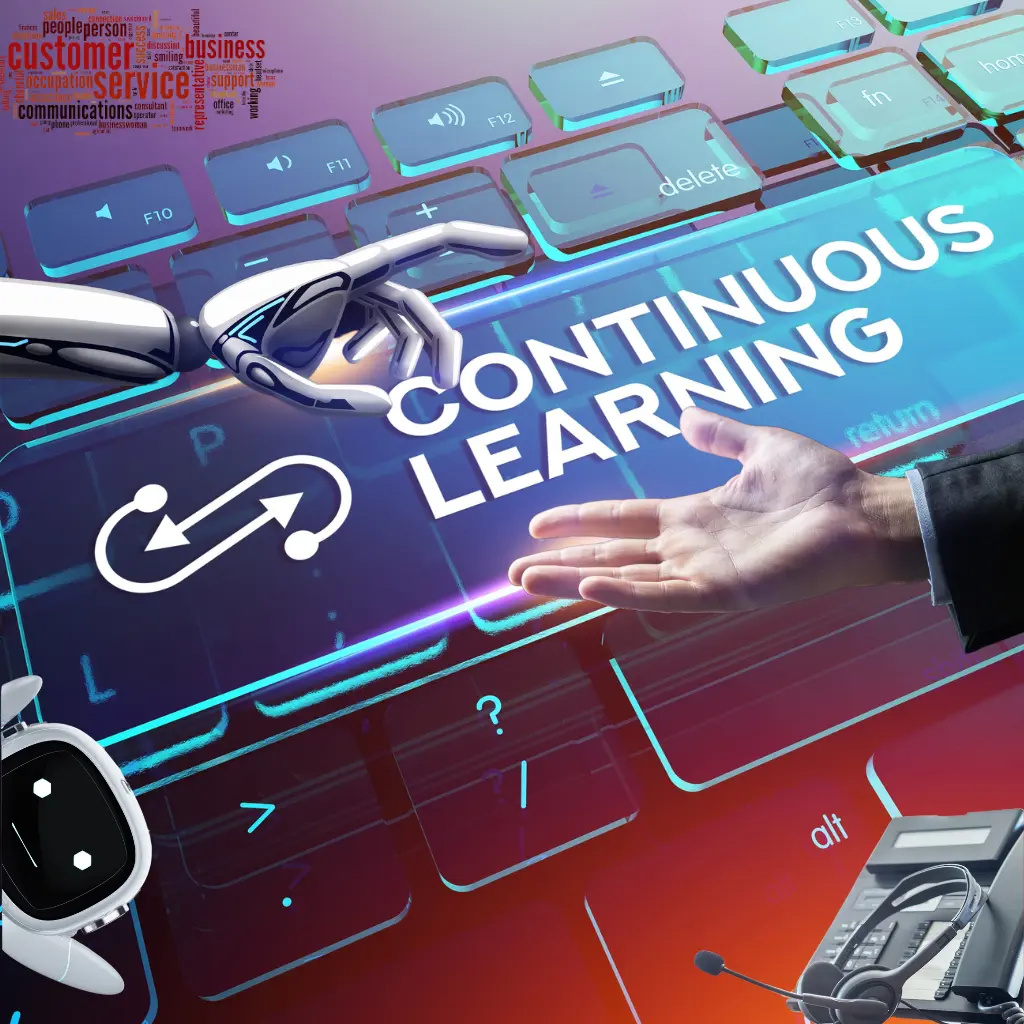
5 Customer Service Tools for Continuous Learning and Improvement
Explore the transformative potential of Customer Service Tools for Continuous Learning and Improvement. This discussion delves into the essential components: business knowledge, software expertise, hardware requirements, training protocols, integrations, and comparative tools. It offers strategic insights into building and implementing these tools for an adaptive and customer-centric approach in the dynamic realm of customer service.
Conclusion
In conclusion, integrating AI Tools for the Customer Services Domain is a pivotal strategy for enhancing customer service operations’ efficiency, personalization, and security. The comprehensive suite of tools discussed, spanning natural language processing, speech recognition, predictive analytics, automation, knowledge management, social media monitoring, facial recognition, data security, and continuous learning, collectively underscores the transformative potential of artificial intelligence.
By leveraging these tools, businesses can streamline customer interactions, tailor services based on individual preferences, and proactively address customer needs. Incorporating NLP and sentiment analysis facilitates nuanced communication, while speech recognition systems enable seamless phone support. Predictive analytics empowers businesses to forecast demand and preemptively address issues, fostering proactive customer retention efforts.
Moreover, AI-driven automation tools optimize repetitive tasks, ensuring swift resolution of customer issues. Knowledge management systems with AI algorithms facilitate self-service, empowering customers to find information independently. Social media monitoring tools and facial recognition technologies contribute to heightened security and improved engagement on digital platforms.
The emphasis on data security and privacy underscores a commitment to safeguarding customer information, an integral aspect of trust-building in the digital era. Continuous learning tools powered by AI algorithms ensure that training programs evolve with dynamic customer needs, fostering a culture of improvement and adaptability.
In navigating the realm of AI Tools for the Customer Services Domain, a strategic approach involving a judicious mix of building from scratch, buying from the market, and customization emerges as the most pragmatic choice. This approach expedites implementation and harnesses the proven capabilities of existing tools, allowing businesses to stay at the forefront of innovation while meeting their unique operational requirements. Integrating AI tools in customer service is a customer-centric approach in an ever-evolving landscape.
Related Articles
- 5 Facial Recognition and Emotion Analysis Tools of Customer Service
- 5 Customer Service Speech Recognition and Processing Tools
- 5 Customer Service Predictive Analytics and Recommendation
- 5 Customer Service Tolls for Automation and RPA
- 5 Customer Service Natural Language Processing (NLP) Tools
- 5 Customer Service Tools for Knowledge Management
- 5 Customer Service Tools for Continuous Learning and Improvement
- 5 Customer Service Tools for Customer Survey Analysis
- 5 Customer Service Tools for Social Media Monitoring
- 5 Customer Service Tools for Data Security and Privacy