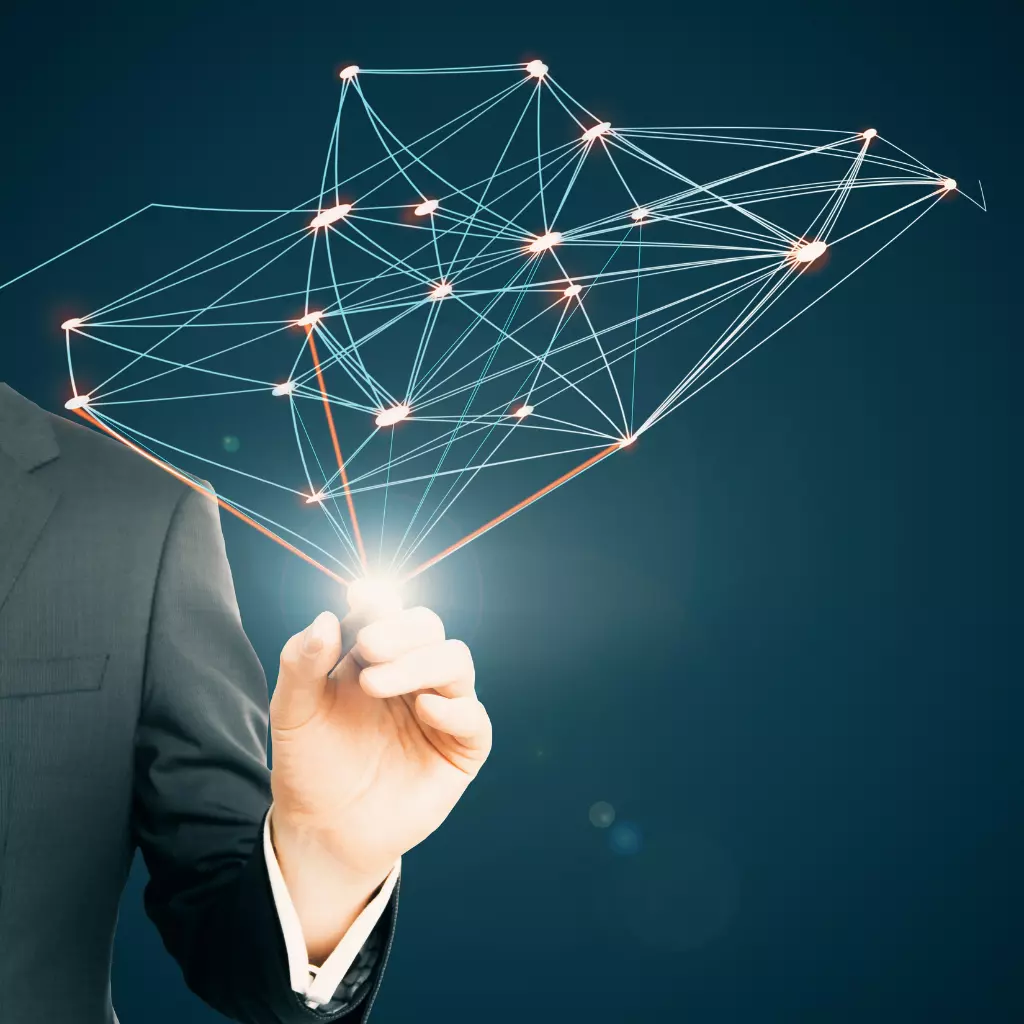
TechInfra in IPA - Intelligent Process Automation
A Comprehensive Guide to Sustainable Implementation
In today’s fast-paced technological landscape, incorporating Intelligent Process Automation (IPA) can bring about transformative organizational efficiency and innovation changes.
This comprehensive guide delves into key facets crucial for sustainable implementation, navigating through the intricate realms of Robotic Process Automation (RPA), Machine Learning (ML) integration, Artificial Intelligence (AI) architecture, Natural Language Processing (NLP), data integration, API connectivity, cloud adoption, security measures, legacy system compatibility, and continuous monitoring.
From strategic decision-making to ethical governance, this guide provides actionable insights, highlighting innovative approaches and best practices. Whether you are embarking on IPA implementation or seeking to optimize existing systems, this guide is an invaluable resource, unlocking the full potential of intelligent automation in today’s dynamic business landscape.
Table of Contents
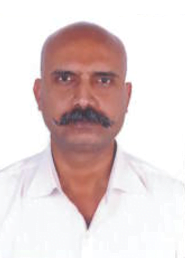
Arindam Roy
An Automation Consultant with 25+ years of IT Experience
Robotic Process Automation (RPA) Implementation
Embarking on a successful Robotic Process Automation (RPA) journey demands a strategic approach encompassing various crucial facets. The foundation lies in the meticulous selection of RPA tools and platforms, a process that involves aligning organizational needs with the capabilities of available tools.
This selection is integral to seamless integration with existing systems, requiring dynamic strategies to harmonize the RPA framework with diverse landscapes. Innovative approaches to RPA process design and automation scripting contribute to a streamlined and efficient automation workflow.
As organizations scale, scalability considerations become paramount in RPA solution architecture. Implementing robust frameworks involves comprehensive testing to ensure reliability, with version control and lifecycle management playing pivotal roles in maintaining system integrity. Balancing human oversight and unattended RPA workflows is critical for optimal efficiency, accompanied by proactive error handling and exception management to preemptively address potential issues.
Moreover, strategic data governance is essential to uphold security and compliance standards. RPA implementations require continuous improvement protocols for iterative enhancements, emphasizing the ever-evolving nature of automation solutions. A successful RPA implementation involves a delicate orchestration of strategic tool selection, integration, scalability planning, and a commitment to continuous refinement and governance.
Machine Learning (ML) Integration
In Intelligent Process Automation, Machine Learning (ML) integration is a cornerstone for advanced data analysis and decision-making. This comprehensive exploration entails a holistic incorporation of ML algorithms into business processes, utilizing their analytical prowess to extract meaningful insights. End-to-end ML model training and deployment strategies are pivotal, ensuring seamless integration into operational workflows.
Continuous learning frameworks form the bedrock of adaptive ML systems, facilitating real-time adjustments and improvements. The dynamic integration of diverse ML technologies amplifies the capabilities of intelligent automation, emphasizing flexibility in adopting evolving methodologies. Real-time model evaluation and optimization present innovative approaches, enhancing the efficiency of ML applications.
Strategic Human-in-the-Loop (HITL) integration becomes crucial for ML-enhanced decision-making, bridging the gap between machine intelligence and human expertise. Proactive data labelling and annotation contribute to robust model performance, while ethical considerations drive the detection and mitigation of biases in ML integration.
Ensuring scalability and efficiency in ML-driven Intelligent Process Automation is paramount, and comprehensive governance protocols are necessary for the entire ML model lifecycle management. This exploration unravels the multifaceted landscape of ML integration, showcasing its transformative potential in shaping the future of intelligent automation.
Artificial Intelligence (AI) Architecture
A robust Artificial Intelligence (AI) architecture is pivotal for organizations navigating the transformative landscape of intelligent technologies. This exploration begins with the strategic design and implementation of comprehensive AI architectures, setting the stage for enhanced business processes. The end-to-end integration of AI components is crucial, ensuring seamless collaboration and synergy between technology and operations.
Innovative AI infrastructure design takes centre stage, emphasizing adaptability and scalability. Holistic frameworks for AI-driven decision support systems contribute to informed, data-driven strategic choices. The dynamic integration of diverse AI technologies, including computer vision and speech recognition, broadens the spectrum of capabilities in intelligent workflows.
Strategic data flow and processing architectures are crucial in optimizing AI-enhanced workflows, fostering efficiency and reliability. Governance protocols are essential to ensure ethical and transparent AI decision-making, accompanied by proactive security measures to safeguard sensitive data and models. Balancing automation with human oversight becomes a delicate art, maximizing the strengths for optimal outcomes in AI-enabled business processes.
Continuous improvement and adaptation protocols are imperative, acknowledging the evolving nature of AI architectures. This holistic journey through AI architecture unveils its transformative potential, laying the groundwork for organizations to thrive in the era of intelligent automation.
Natural Language Processing (NLP) Integration
Natural Language Processing (NLP) integration stands at the forefront of revolutionizing human-computer interactions, exploring linguistic nuances and communication modalities. The strategic implementation of NLP for text and speech processing is foundational, enabling machines to comprehend and respond to human language effectively. This integration extends to enhancing human-bot interaction, ensuring a seamless and natural exchange of information.
Addressing language variations and nuances is a dynamic aspect of NLP integration, requiring innovative approaches to capture the intricacies of diverse linguistic expressions. NLP analysis goes beyond surface-level understanding, employing techniques to extract meaningful insights from textual and verbal data. The holistic integration of NLP in document processing and information extraction amplifies its utility across various domains.
Governance protocols play a vital role in ensuring ethical and inclusive NLP integration practices, addressing concerns related to biases and fairness. Proactively considering multilingual challenges is essential, fostering a user-centric design that prioritizes inclusivity and enhances user experience. Adaptive NLP models, designed for continuous learning, contribute to improved language understanding over time.
Security measures are paramount in NLP integration, safeguarding sensitive textual and verbal data. This exploration of NLP integration unveils its transformative potential, showcasing its ability to bridge the gap between human language and machine understanding while emphasizing ethical considerations and user-centric design principles.
Data Integration Strategies
Effective data integration is pivotal for harnessing the full potential of intelligent automation. Organizations must synchronize data across various systems to ensure smooth and efficient information flow. It is achieved by keeping the data consistent and up-to-date across all platforms.
By doing so, everyone in the organization can access the same information anytime. This process is the backbone of the operation. Holistic data integration frameworks play a vital role in ensuring that data moves effortlessly, breaking down silos and facilitating a comprehensive understanding of organizational information.
Data cleansing and normalization techniques are instrumental in maintaining the quality and consistency of integrated data. Dynamic data mapping strategies contribute to accurate integration, ensuring data is interpreted consistently across different systems. A paramount concern is ensuring data integrity and security in cross-system data flows, with robust measures in place to protect sensitive information.
Innovative approaches to real-time data integration add agility to organizational processes, allowing for timely decision-making. Striking a balance between centralized and decentralized data integration architectures is crucial, aligning with the organization’s needs.
Governance protocols are essential for ethical and transparent data integration practices, including considerations for data quality measures. Proactively addressing scalability and efficiency concerns ensures that data integration evolves seamlessly with the organization’s growth and technological advancements, supporting the broader goals of intelligent automation.
API Connectivity and Management
API Connectivity and Management are the linchpins in orchestrating seamless interactions between systems, playing a pivotal role in the landscape of Intelligent Process Automation.
The strategic development and implementation of robust API connectivity solutions are fundamental, ensuring that disparate systems communicate cohesively. Holistic API management is imperative for addressing security and scalability concerns, underlining the need for a comprehensive approach that optimizes performance.
Establishing API connections requires meticulous integration strategies, fostering interoperability across diverse platforms. Balancing API versioning and backward compatibility is a nuanced aspect, demanding innovative approaches to ensure smooth transitions without disrupting existing functionalities. Access control and identity management become critical components in securing API interactions.
API lifecycle management requires dynamic handling to meet evolving organizational needs, from creation to deprecation. Compliance with data privacy regulations is paramount, emphasizing governance protocols for ethical and transparent API management practices. Proactive monitoring and troubleshooting mechanisms enhance the reliability of API integration workflows, ensuring quick resolution of potential issues.
Scalability considerations round off the spectrum, acknowledging the ever-growing demands of intelligent process automation. This exploration underscores the multifaceted nature of API connectivity and management, delineating its critical role in fostering efficient, secure, and scalable interactions within the technological ecosystem.
Cloud Integration and Migration
Intelligent Process Automation (IPA) has evolved due to the strategic integration of cloud infrastructure. This connection is essential to understand the benefits of IPA. This pivotal shift necessitates comprehensive cloud migration strategies, especially when transitioning from on-premise environments. Adopting cloud-based storage and computing introduces innovative considerations, emphasizing the need for scalable and efficient solutions tailored for IPA.
Balancing security and compliance is paramount in cloud integration and migration, with governance frameworks guiding ethical and transparent practices. Dynamic scalability protocols are integral, ensuring that cloud-enabled IPA solutions can flexibly adapt to varying workloads. Proactive data migration planning becomes crucial for a seamless transition, emphasizing the importance of data availability and accessibility in cloud-integrated workflows.
User-centric design principles drive enhanced experiences in cloud-enabled environments, optimizing user interactions. Continuous monitoring and optimization protocols further solidify the reliability and efficiency of cloud-integrated IPA environments, aligning with the evolving demands of intelligent automation.
In essence, this exploration delves into the multifaceted landscape of cloud integration and migration in the context of IPA. It underscores the strategic decisions, innovative considerations, and ethical frameworks that organizations must navigate to unlock the full potential of intelligent automation in a cloud-centric era.
Security Measures in IPA
Security is paramount in Intelligent Process Automation (IPA), demanding a multifaceted approach to safeguard automation workflows. To keep sensitive information from being accessed by unauthorized parties, it’s essential to take proactive steps to encrypt data and control access.
This is the foundational layer of data security. Comprehensive security protocols are imperative for secure data transmission within automation workflows, emphasizing the need for robust measures to prevent data breaches.
Dynamic identity management strategies are pivotal in safeguarding IPA environments, ensuring only authorized personnel access critical systems. Governance frameworks guide ethical and transparent security practices, aligning security measures with organizational values. Innovative approaches to regulatory compliance are integral to secure IPA implementations, ensuring adherence to industry standards and legal requirements.
Holistic threat detection and response protocols are essential for IPA security, enabling organizations to address and mitigate potential risks swiftly. Balancing security with user accessibility becomes a delicate task, emphasizing the importance of user-friendly yet secure IPA systems. To stay safe from the constantly changing threats in the world of automation, it’s essential always to keep an eye on security and adjust your approach accordingly.
Data privacy measures are implemented to safeguard sensitive information handled by IPA processes. This helps reinforce trust in automation solutions. Collaborative security protocols for human-bot interaction contribute to secure data handling and foster a safe automation environment. This comprehensive security approach underscores the commitment to maintaining data integrity, confidentiality, and availability in IPA ecosystems.
Legacy System Compatibility
Integrating Intelligent Process Automation (IPA) with legacy systems requires a strategic and comprehensive approach. Strategic integration frameworks are pivotal in establishing seamless interaction with legacy systems and fostering interoperability between old and new technologies. Holistic legacy system modernization approaches ensure compatibility with IPA, facilitating a smooth transition towards more advanced automation solutions.
Dynamic data flow protocols become essential for maintaining connectivity between legacy and modernized systems. Innovative strategies focus on preserving legacy system functionality within IPA, acknowledging the importance of retaining critical processes. Governance protocols guide ethical and transparent practices, aligning legacy system compatibility with organizational values.
Proactively identifying and mitigating integration challenges is crucial for successful interaction with legacy systems. Balancing legacy and modernized infrastructure is critical for optimal IPA performance, ensuring a harmonious coexistence. Continuous monitoring and adaptation protocols are necessary to address evolving compatibility issues and maintain the effectiveness of the integrated ecosystem.
User-centric design principles contribute to a smooth interaction between users and legacy systems, enhancing the overall user experience during integration. When adding old systems to a modern computer environment, it’s essential to take strong measures to protect sensitive data and processes.
This includes maintaining confidentiality and ensuring integrity. This comprehensive approach ensures a synergistic integration between IPA and legacy systems, respecting the past while paving the way for future automation advancements.
Continuous Monitoring and Maintenance
Continuous monitoring and maintenance form the backbone of a resilient and efficient Intelligent Process Automation (IPA) ecosystem. A strategic implementation of constant monitoring frameworks for IPA infrastructure is foundational, ensuring a proactive approach to system health. Proactive error detection and resolution protocols are crucial, preventing potential issues from escalating and impacting automation workflows.
Holistic maintenance strategies are imperative for sustaining optimal performance in IPA systems. Dynamic version control and lifecycle management ensure that IPA components evolve in a controlled and efficient manner. Real-time monitoring of process flows, and automation scripts introduce innovative approaches, allowing for immediate insights and adjustments.
Balancing automation with human oversight is a nuanced aspect of continuous monitoring, acknowledging the complementary roles of technology and human expertise. Governance protocols guide ethical and transparent continuous monitoring practices, aligning with organizational values. User-centric design principles enhance the monitoring experience, making it intuitive and user-friendly.
Keeping sensitive data secure is crucial during continuous monitoring. Having appropriate security measures in place is essential for maintaining the confidentiality and integrity of information. It helps to prevent unauthorized access and protect against potential threats and risks.
Adaptive protocols for iterative enhancements, based on constant monitoring insights, contribute to the evolution and improvement of IPA technology over time. This comprehensive approach to continuous monitoring and maintenance establishes a robust foundation for sustained performance, security, and user satisfaction in the dynamic landscape of Intelligent Process Automation.
Conclusion
In conclusion, this comprehensive guide underscores the significance of strategic decision-making and ethical governance in navigating the multifaceted landscape of Intelligent Process Automation (IPA).
From Robotic Process Automation (RPA) to Machine Learning (ML) integration, Artificial Intelligence (AI) architecture, Natural Language Processing (NLP), data integration, API connectivity, cloud adoption, security measures, legacy system compatibility, to continuous monitoring, each facet plays a crucial role in optimizing technological infrastructure for sustainable implementation.
As organizations embark on the journey of IPA, the seamless integration of innovative approaches, user-centric design principles, and adaptive protocols for continuous enhancement are paramount. Balancing automation with human oversight, ensuring data integrity, and prioritizing security measures contribute to the robustness of the IPA ecosystem. The guide emphasizes the strategic adoption of cloud infrastructure, dynamic data integration strategies, and the importance of maintaining compatibility with legacy systems.
In this dynamic business landscape, where technological evolution is constant, staying at the forefront of intelligent automation requires continuous learning and adaptation. Organizations that implement these insights into their IPA processes will witness enhanced efficiency and scalability and foster a culture of innovation.
By optimizing technological infrastructure, embracing ethical practices, and prioritizing user experience, organizations can truly unlock the transformative potential of Intelligent Process Automation.
Related Articles
- Choosing the Right Automation Tool in 7 Steps
- A 9-Step Guide to IPA Implementation: Energise Your Operations
- Navigating the Roadblocks of IPA – The Top 9 Challenges
- AI in Intelligent Process Automation – Unleash the Power of AI
- 9 IPA Examples in Small-Scale Industries
- Power of Intelligent Business Process Automation – Efficiency
- AI Business Process Management: Unleashing the Power
- Business Process Management with AI Integration
- AI for Reengineering Business Processes
- Hyperautomation: Redefining BPM with AI
- The Role of AI in Business Process Modelling
- AI-driven Customer Onboarding – Unleash the Power of AI
- Effective Process mapping in Intelligent Process Automation
- Intelligent Process Automation Adoption – The Best Strategy Guide
- TechInfra in IPA: A Comprehensive Guide
- Elevating Intelligent Automation with Continuous Improvement
- Comprehensive Approach to Cost-Benefit Analysis in IPA
- Vendor Selection in IPA – Comprehensive Guide
- Implementing Agile in Intelligent Process Automation (IPA)
- Scalability and Integration in Intelligent Process Automation
- Cognitive Automation in IPA: Innovating Ethical Efficiency
- Mastering Symphony: Bot Development in IPA Unveiled
- UI/UX Mastery in IPA: Elevating Automation Experiences
- Compliance and Security in IPA – A Guide Ensuring Trust
- Impact of Performance Monitoring and Analytics in IPA
- Driving Success: Data Management in IPA
- Crucial Role: Documentation in IPA Triumph
- Optimizing IPA – Continuous Improvement Strategies