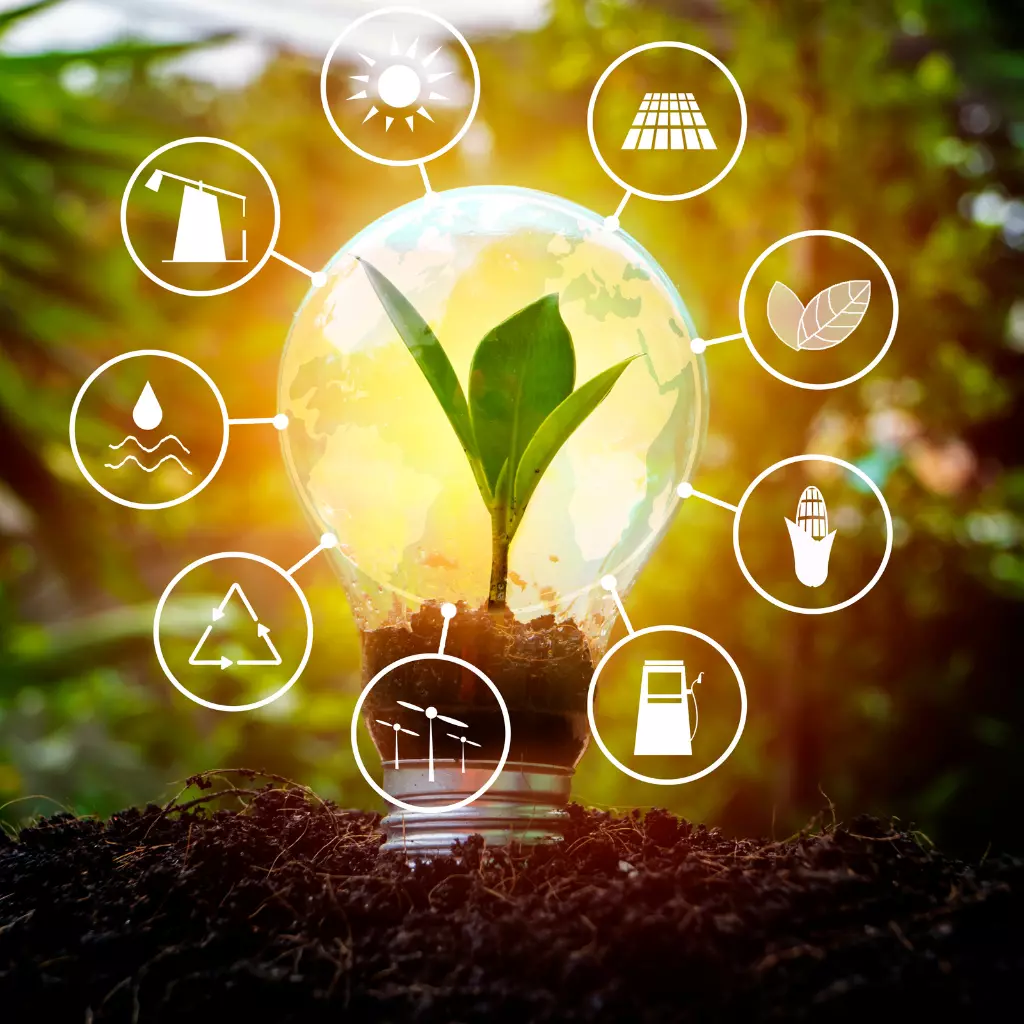
From Solar to Wind
AI in Renewable Energy Forecasting
As the global push towards sustainability intensifies, Artificial Intelligence (AI) is becoming a game-changer in the energy sector.
This article delves into the Impact of AI on Renewable Energy forecasting, from solar energy prediction to wind power forecasting and demand prediction. We’ll explore how sophisticated machine learning techniques contribute to grid balancing and energy consumption analytics.
Prepare for a thrilling insight into the future of energy management, driven by integrating AI and renewable energy.
Table of Contents
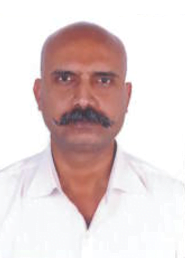
Arindam Roy
An Automation Consultant with 25+ years of IT Experience
Shining a Light: Solar Energy Predictions with AI
Prepare to be blown away as the future of renewable energy gets a game-changing boost from Artificial Intelligence (AI)!
Get ready to witness a complete transformation in how we harness the sun’s power!AI enables more intelligent, efficient solar energy management by predicting energy production. It uses a range of data, such as past weather patterns, real-time sun trajectories, and geographical location, to accurately forecast the yield of solar panels.
This prediction aids in optimizing power grids and managing supply chains effectively. AI can also identify mechanical issues in solar systems before they become problematic, minimizing downtime, reducing costs, and ensuring maximum productivity.
In essence, AI is key to unlocking the full potential of solar energy.
Gusts & Gigawatts: Wind Power Forecasting through AI
Harnessing the unpredictable nature of wind to generate power requires precise forecasting, which is critical for efficient energy distribution and grid stability. This is where AI plays a significant role.
Using complex algorithms and machine learning, AI can analyze large datasets of historical wind speed, direction, temperature, and topographic details to generate accurate wind power forecasts.
These AI models can also learn from real-time data, improve their predictions over time, and forecast weather changes with greater accuracy, an essential aspect of wind power generation.
Thus, AI in wind power forecasting equates to increased renewable power efficiency, reinforcing our transition towards sustainable energy.
Powering Predictions: AI in Energy Demand Forecasts
AI is revolutionizing energy demand forecasts. With the world transitioning towards sustainable energy, predicting consumption levels with precision is critical to optimizing resource allocation and preventing power outages.
Traditional forecasting methods, reliant on historical trends, often need to catch up on capturing the dynamic nature of energy consumption. With its rich datasets and predictive capabilities, AI helps craft accurate, real-time forecasts.
It uses machine learning algorithms to analyze past data and identifies nonlinear relationships and seasonality patterns that impact demand. AI also considers weather or public events that influence usage, allowing energy providers to meet fluctuations effectively.
Hence, AI in energy forecasting creates a sustainable, efficient, and reliable energy infrastructure.
Balancing Act: Machine Learning for Stable Grids
Machine learning can offer an effective balancing act for maintaining stable grids. As the energy sector advances, it involves numerous intermittent renewable energy sources, which brings volatility to the power grid.
The challenge is to balance the supply and demand efficiently constantly. Here, machine learning algorithms can analyze power production and consumption patterns, predict grid fluctuations and help in enhancing grid stability.
These predictive models allow utilities to manage grid operations, minimize downtime and improve reliability proactively. They can also optimize energy storage systems, contributing to a balanced, stable, and resilient grid.
Thus, machine learning technology plays an integral role in modern grid management.
Decoding Consumption: AI-Driven Energy Analytics
AI-driven energy analytics allows for detailed decoding of consumption patterns. This data-centric approach helps understand the intricacies of energy usage and minimize waste.
Machine learning algorithms can predict energy demand and supply by analyzing consumption metrics and facilitating efficient resource management. Utilities can leverage this data to implement dynamic pricing, incentivize off-peak usage, and reduce carbon footprints.
Moreover, consumers can receive personalized energy reports, providing insights into their behaviour and suggesting improvements. These advanced analytics empower people and organizations to make informed, sustainable choices, fostering a culture of energy conservation and boosting eco-efficiency.
AI in energy analytics is undoubtedly shaping a green future.
Harnessing Peaks and Troughs: Dynamic AI Energy Modelling
Harnessing peaks and troughs refers to leveraging energy production’s natural highs and lows. With the integration of AI, the task becomes more efficient. Dynamic AI energy modelling enables predictive analytics, showing possible areas of energy inefficiency and suggesting enhancements.
It considers various factors such as weather conditions, equipment state, and performance to manage energy flow efficiently. The technology’s ability to adapt and optimize energy usage helps harness renewable energy sources, such as solar and wind, by predicting their output and ensuring optimum utilization.
It aids in reducing energy waste and contributes to creating a more sustainable future.
Grid Guardians: AI in Power Infrastructure Management
Integrating AI in managing power infrastructures is revolutionizing the energy sector. The technology aids in predictive maintenance, thus reducing the risk of sudden outages. AI models help forecast energy generation and demand, optimizing grid performance.
The technology enhances system reliability by identifying potential threats and preventing infrastructural damage. AI can also help automate grid responses, effectively managing supply during peak demand.
Thus, with “Grid Guardians,” we can ensure efficient power distribution, reduce energy wastage, and proactively foresee and fix grid faults, leading to more robust and resilient power systems.
From Sun to Socket: Optimizing Renewable Energy Output
Optimizing renewable energy output from the sun to socket involves perfecting the systems used in harnessing and channelling clean energy to households.
Solar panels, for instance, need to be positioned for maximum exposure to sunlight. More innovations are also emerging, including solar tracking systems that align panels to the sun’s movement.
Regarding transportation, it’s important to minimize energy loss in transmission lines. Localizing energy sources can reduce the distance between production and consumption, improving efficiency.
Systems like energy storage or distributed energy grids can store surplus power during peak production times, ensuring a steady energy output even when conditions are less optimal.
Future-Ready: Adapting Energy Models with AI
The role of AI in shaping future-ready energy models is indispensable. AI optimizes existing energy systems and changes how energy is stored, transmitted, and used.
It aids in monitoring energy consumption patterns, predicting energy demands, and automating energy distribution systems. AI-driven analytics enable precise predictions and effective decision-making, ensuring optimal utilization of resources.
Moreover, it plays an integral role in the transition towards renewable energy sources, managing their intermittent nature and ensuring a steady and reliable energy supply.
Thus, we are shaping a more sustainable and resilient energy future by incorporating AI into energy models.
Energizing Insights: AI-Driven Strategies for Renewable Energy
AI-driven strategies have revolutionized the renewable energy sector by providing energizing insights for optimizing resources. AI systems can forecast energy generation where inconsistency is common, such as in solar and wind power.
They analyze climatic data to predict output levels, facilitating optimal energy usage and reducing wastage. AI also supports the predictive maintenance of renewable energy systems by identifying potential failures before they occur.
This not only boosts efficiency but also prolongs lifespan and profitability. Furthermore, AI algorithms could play an integral role in managing and distributing power in grid systems, preventing blackouts.
These solutions underline the potential of integrating AI with renewable energy strategies.
Conclusion
The blend of AI and renewable energy is shaping an exciting future for the energy sector. With predictive analytics, real-time data analysis, and sophisticated algorithms, AI redefines how we produce, consume, and manage energy.
From optimizing solar and wind energy output to forecasting demand, operating grids, and facilitating predictive maintenance, AI is at the forefront of maximizing renewable energy’s potential.
Moreover, the impact of AI continues beyond efficiency and optimization. It catalyzes fostering a culture of sustainability and informed decision-making. This symbiotic relationship between AI and renewable energy not only promises a future with reduced carbon footprints and cleaner energy but also drives innovations that could redefine the very landscape of the energy sector.
Embracing AI is an option and a necessity as we strive for a greener, more sustainable, and energy-efficient future.
Related Articles
- Revolutionizing Energy Management: How AI Boosts Efficiency and Sustainability
- Beyond Conventional Power: AI in Smart Grids and Infrastructure Innovations
- From Drill Bits to Data Bits: AI in Oil and Gas Operations
- Atomic Intelligence: AI in Nuclear Energy Safety and Management
- AI in Various Industry Sectors