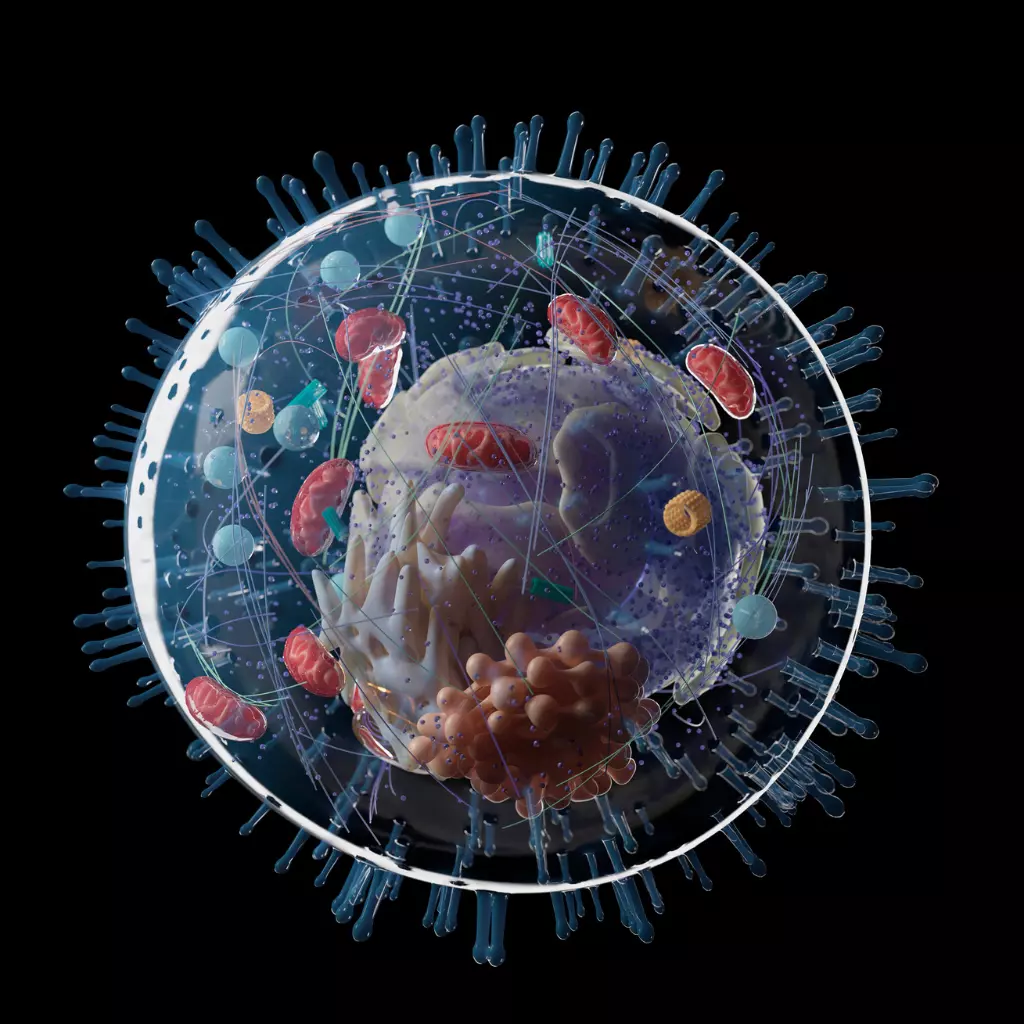
Predicting and Controlling Outbreaks
AI in Epidemiology
In the expansive world of healthcare, the intersection of AI in epidemiology heralds a new dawn. As diseases evolve, so too must our methodologies.
Enter Artificial Intelligence—a catalyst transforming our approach from tracking infectious diseases to pioneering advanced vaccine development.
This article embarks on a journey, unveiling how AI is not just predicting disease outbreaks but also reframing our understanding of transmission dynamics.
Alongside this, we’ll touch upon the pivotal role of data sources in this nexus and navigate the ethical terrains of an AI-integrated future. Join us as we chart the course of epidemiology, driven by the power and promise of AI.
Table of Contents
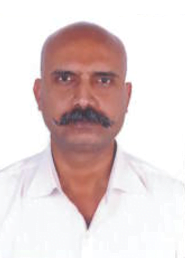
Arindam Roy
An Automation Consultant with 25+ years of IT Experience
Modern epidemiology and AI
The world of healthcare has always been dynamic, with epidemiology at its forefront. But with the integration of AI in epidemiology, the field has gained unprecedented momentum. Gone are the days when tracking infectious diseases required manual data inputs and days of analysis. Today, AI in infectious disease tracking offers real-time insights, pinpointing outbreaks with impressive accuracy.
Predicting disease outbreaks used to be more of an art based on historical data and patterns. Now, with AI’s ability to analyze vast amounts of data quickly, predicting disease outbreaks is more science than guesswork. The impact doesn’t stop there. AI in vaccine development has expedited research, with algorithms analyzing potential vaccine compounds in a fraction of the time humans would require.
But what about the broader spectrum of disease transmission? Analyzing disease transmission patterns with AI tools helps researchers understand where a disease might appear next and why and how. This knowledge is pivotal for AI-driven public health decisions, optimizing interventions and resource allocation for maximum effect.
Data undoubtedly, fuels these advancements. Data sources and AI in epidemiology provide a symbiotic relationship where each strengthens the other. But as we delve deeper into this relationship, we must remain vigilant. Ethical concerns in AI-driven epidemiology are ever-present, urging transparency and accountability.
How does all this impact the economy and the training of future experts? The economic implications of AI in epidemiology and the need for training epidemiologists for the AI era cannot be overstated.
As we journey through these intersections of technology and health, one wonders: What could be the next monumental shift catalyzed by AI in disease control?
Predictive models for outbreaks
The fight against infectious diseases hinges on one crucial component – foresight. AI in epidemiology has been a game-changer in this age, transforming how we predict potential outbreaks. At its core, AI’s prowess in predicting disease outbreaks lies in its ability to sift through enormous datasets, discern patterns, and forecast risks with heightened accuracy.
With AI in infectious disease tracking, we now harness real–time data from various sources– from hospitals to social media. This vast data influx is processed instantaneously, pinpointing disease hotspots before they spiral out of control.
Yet, it’s not just about recognizing the emergence of a disease; it’s about understanding its trajectory. Analyzing disease transmission patterns becomes infinitely more insightful with AI. By integrating travel data, environmental factors, and sociodemographic shifts, AI crafts intricate models that showcase where a disease might strike next and how fast and comprehensively it might spread.
AI-driven public health decisions lean heavily on these predictive models. With them, authorities can allocate resources more effectively, institute quarantines promptly, or even guide AI in vaccine development by highlighting vulnerable populations.
However, while AI’s capabilities in this arena are expansive, they’re challenging. To achieve practical epidemiology, data sources and AI must align seamlessly. The ethical concerns surrounding AI-driven epidemiology are greatly magnified when predictive models influence large-scale health directives, making it crucial to take a firm stance on upholding ethical practices.
Facing such transformative power in predicting outbreaks, one can’t help but ponder: How might AI further refine these models in the ever-evolving landscape of infectious diseases?
Speeding up vaccine research
Vaccine development, traditionally, has been a laborious journey spanning years, even decades. Yet, with the advent of AI in epidemiology, the horizon of vaccine research has seen transformative acceleration. As global health challenges mount, this rapidity isn’t just desirable – it’s indispensable.
Diving into AI in vaccine development, one recognizes its innate ability to scan and analyze vast molecular databases. AI can predict how different compounds might interact with specific pathogens by doing so. Instead of trial-and-error approaches, researchers can prioritize compounds that AI models deem most promising. This predictive prowess of AI in infectious disease tracking offers a tangible edge in the early stages of vaccine formulation.
Moreover, AI further refines its models as we gather data about infections and patient responses. The loop of analyzing disease transmission patterns, understanding host responses, and iterating vaccine candidates becomes faster and more precise. It’s no wonder that AI-driven public health decisions are increasingly intertwined with these advanced research methodologies.
However, as with any powerful tool, challenges persist. Data sources and AI in epidemiology must remain consistent and robust. Any oversight or inaccuracy can skew results, emphasizing the weight of ethical concerns in AI-driven epidemiology.
Navigating this intricate dance of speed, precision, and responsibility, the role of AI in vaccine research is undeniably transformative. But as we push the boundaries of rapid vaccine development, the question lingers: How can we ensure that the speed doesn’t compromise the essential tenets of safety and efficacy?
Understanding transmission dynamics with AI
When battling infectious diseases, understanding transmission is half the battle won. Dive deep into the heart of AI in epidemiology, and you’ll find intricate models mapping out how pathogens spread within communities. These insights, born from cutting-edge AI technologies, are reshaping our knowledge of disease dynamics.
In the earlier stages of outbreaks, predicting disease outbreaks based on transmission patterns heavily relied on historical data and limited real-time inputs. Now, the integration of AI in infectious disease tracking fetches data from diverse sources, ranging from hospital records to travel histories and even climate data. When fed into AI algorithms, these elements yield spread patterns with astonishing precision.
Analyzing disease transmission patterns is not just pinpointing the ‘where’, ‘how’, and ‘why’. Factors such as population density, social interactions, and local cultural practices can all impact the spread of a disease. Therefore, AI-powered public health decisions are based on numerical data and a thorough understanding of socio-environmental complexities. Let me know if you need anything else.
However, these data-intensive models necessitate impeccable data sources and AI in epidemiology synchronization. The significance of accuracy and ethical concerns in AI-driven epidemiology is heightened as we rely heavily on AI insights for vital public health directives. It’s not merely about data; it’s about human lives, communities, and global health trajectories.
As we unravel the complex tapestry of transmission dynamics aided by AI, it beckons the query: In a world where human interactions and environments are ever-evolving, how might AI continue to refine its understanding of these intricate dynamics?
Data-driven public health strategies
In the labyrinth of global health challenges, data emerges as the North Star guiding interventions and decisions. AI in epidemiology is at the centre of this revolution, reshaping public health strategies with precision and adaptability.
Traditionally, public health strategies were driven by generalized insights. Today, AI in infectious disease tracking provides granularity. Every case, interaction, and symptom reported becomes valuable data points. This rich data fabric allows for predicting disease outbreaks with astounding accuracy, enabling proactive rather than reactive responses.
But beyond mere prediction lies the power of understanding. Analyzing disease transmission patterns helps public health officials to discern hotspots, vulnerable communities, and potential super-spreader events. When these insights drive policy, we witness AI-driven public health decisions that are both effective and targeted.
Still, there’s an intricate balance to be maintained. While data sources and AI in epidemiology provide a formidable alliance, the weight of responsibility is profound. Misinterpreted or misused data can lead to dire consequences, magnifying the importance of addressing ethical concerns in AI-driven epidemiology. Every strategy every directive, impacts real lives and communities.
Moreover, the vast potential of AI in shaping public health also underscores the need for training epidemiologists for the AI era. They must navigate this data-rich landscape with both technological proficiency and ethical integrity.
Given this pivotal role of data in shaping health futures, one can’t help but wonder: As data sources continue to expand and diversify, how will AI further tailor public health strategies to meet unique community needs?
Sourcing and managing epidemiological data
In the age of information, data is more than just a valuable resource; it’s the lifeblood driving impactful insights. How this data is sourced and managed can make all the difference in the war against infectious diseases.
A diverse array of platforms contributes to AI in infectious disease tracking. Traditional hospital and clinic records meld with newer, innovative sources like social media sentiment analysis, wearable health devices, and satellite imagery. Each source brings its unique perspective, enriching the understanding of disease spread and predicting disease outbreaks with more nuance.
Yet, with this vast influx comes the challenge of management. Analyzing disease transmission patterns requires extended data sets and clean, reliable, harmonized data. Standardizing information from varied sources and ensuring accuracy is pivotal for effective AI-driven public health decisions.
This mammoth task of data management isn’t just technical but also ethical. Given the sensitive nature of health data, ensuring privacy and confidentiality becomes paramount. As we dive deeper, ethical concerns in AI-driven epidemiology surface, emphasizing the significance of transparent, secure, and consent-driven data practices.
Amid this dynamic interplay of sourcing and managing data, the collaboration of tech experts and epidemiologists becomes crucial. After all, training epidemiologists for the AI era involves imbuing them with data literacy alongside their medical expertise.
With such intricate layers to data sourcing and management, a pertinent question arises: How can we further fortify our epidemiological data’s reliability and ethical integrity in the AI age?
Addressing ethical dilemmas
The fusion of AI in epidemiology promises unprecedented advancements, yet it comes with its ethical quandaries. In the whirlwind race of harnessing data for health benefits, where do we draw the line on privacy, consent, and transparency?
Central to AI in infectious disease tracking is gathering personal health data. While these datasets are pivotal for predicting disease outbreaks and analyzing disease transmission patterns, there’s an underlying challenge: How do we uphold data protection rights? Every byte of health data is personal, sensitive, and subject to misuse in the wrong hands.
The drive for AI-driven public health decisions further compounds these ethical dilemmas. While AI models are powerful, they can sometimes produce biased or unfair results based on the data they’ve been trained on. Addressing the nuances of representation, equity, and fairness in AI outputs becomes paramount.
Moreover, with varied data sources and AI in epidemiology, the integrity of data collection practices is under scrutiny. Ensuring data is sourced with full consent and transparency is not just a legal requirement but an ethical imperative.
Even as we grapple with these challenges, training epidemiologists for the AI era gains new dimensions. It’s not just about data analytics or AI modelling; it’s about instilling a deep-rooted sense of ethical responsibility.
Amidst these reflections on ethical practice, a pressing question emerges: As we scale the heights of AI’s potential in epidemiology, how do we ensure the foundational ethics of medical practice remain uncompromised and at the forefront?
Training and education for future epidemiologists
The evolving landscape of AI in epidemiology signals a transformative shift in the world of public health. As algorithms and data models become central, epidemiologists’ education and training paradigm is undergoing a radical overhaul.
It’s not just about understanding pathogens or analyzing disease transmission patterns anymore. The modern epidemiologist must be adept at navigating vast datasets, harnessing AI in infectious disease tracking, and discerning how AI models present. This interplay of biology and technology is the new normal.
The curricula of epidemiological studies are thus widening. They’re incorporating courses on data analytics, understanding AI-driven public health decisions, and ethical practices associated with data management. As we fuse classical epidemiological techniques with AI tools, training epidemiologists for the AI era is about amalgamating traditional methods’ wisdom with modern tech’s prowess.
Yet, it’s not solely about skills or techniques. With the rise of ethical concerns in AI-driven epidemiology, imbuing a sense of responsibility and ethics in budding epidemiologists is crucial. They must be trained to question, critique, and seek transparency in the AI models they utilize.
Furthermore, as they interact with diverse data sources and AI in epidemiology, understanding each data source’s nuances, strengths, and limitations is fundamental.
Given this transformative shift in training and education, one ponders: As the next generation of epidemiologists emerges at the intersection of biology and AI, how will their unique skillset reshape the future contours of public health and global disease management?
Case study: AI's role in a recent outbreak
The transformative capabilities of AI in epidemiology came to the limelight during a recent viral outbreak that shook the globe. When a new, unfamiliar pathogen emerged, traditional methods of tracking and understanding its spread seemed daunting. Enter AI.
In the early days of the outbreak, AI in infectious disease tracking systems sounded alarms about unusual pneumonia-like symptoms in a localized area. Before human epidemiologists could recognize the threat, AI had already predicted disease outbreaks across significant transport hubs and densely populated regions.
Advanced algorithms have been utilized to analyze disease transmission patterns by harnessing vast databases. They identified potential super-spreaders and vulnerable groups and predicted hospital overload periods. This real-time analysis equipped governments and health organizations to make swift, AI-driven public health decisions.
Diverse data sources and AI in epidemiology merged, offering a holistic view. Social media sentiment, travel records, and clinical reports collectively informed strategies, while AI models also played pivotal roles in preliminary vaccine research, cutting down months, possibly years, from the R&D process.
Yet, it was challenging. With the rush to utilize AI, some ethical concerns in AI-driven epidemiology emerged, particularly around data privacy and the potential for misinformation.
Reflecting on this case study, the synergy between AI and human expertise was undeniable. However, it also raised questions about our ethical and technological preparedness for future health crises. As we laud AI’s role in managing this outbreak, What lessons have we truly absorbed, and how will they inform our response to the next global health challenge?
Gazing into the future
When we consider the trajectory of AI in epidemiology, the horizon gleams with promise. As data sources burgeon and computational capacities expand, the synergy between AI and epidemiological pursuits only strengthens.
One can envision an era where AI in infectious disease tracking is not merely reactive but proactive. Imagine a world where we can anticipate disease outbreaks before they show symptoms. With the help of massive data streams, this could be a reality in the not-too-distant future.
Moreover, as we venture deeper into understanding pathogens, analyzing disease transmission patterns with AI will likely offer granular insights into predicting individual susceptibilities and treatment responses. Imagine a world where AI-driven public health decisions manage broad populations and tailor strategies for personal health profiles.
Yet, this radiant future isn’t without shadows. As the union of data sources and AI in epidemiology intensifies, so does the responsibility to handle data ethically and securely. Additionally, training epidemiologists for the AI era means a constant, ever-evolving curriculum that marries the best of technology with the deep-rooted ethics of healthcare.
As we stand on the cusp of this AI-driven epidemiological revolution, a lingering thought surfaces: Amidst the dazzling prospects and complex challenges, are we, as a global community, ready to harness AI’s full potential responsibly and equitably in safeguarding our shared health future?
In Conclusion: Embracing the AI-Driven Future of Epidemiology
The journey of AI in epidemiology paints a picture of evolution, bridging traditional methods with groundbreaking technology. AI’s prowess has undeniably reshaped our approach to health crises, from early alarms in infectious disease tracking to predicting disease outbreaks. The dynamic interplay of analyzing disease transmission patterns with machine learning offers insights unparalleled by human analysis alone.
Yet, as with all revolutions, challenges abound. Our dive into data sources and AI in epidemiology highlighted the depth of available data and the accompanying ethical quagmire. While AI-driven public health decisions can streamline processes, data privacy and equity questions loom large. Our responsibility lies in leveraging technology and ensuring its honest and fair application.
The emphasis on training epidemiologists for the AI era signifies a shift in the education landscape, necessitating a blend of biology and tech expertise. As we’ve seen, future epidemiologists are as comfortable with algorithms as pathogens. This synergy promises a holistic approach to disease management that respects historical practices and modern innovations.
The recent outbreak case study has left us all with a lot to ponder, and one thing that stands out is the incredible role played by AI. It’s truly awe-inspiring to see how much of an impact this technology has had on the situation. Yet, the ethical concerns spotlighted the need for caution. As we gaze ahead, the potential of AI in epidemiology is vast, but its responsible use is paramount.
Our shared health future hinges on harmonizing AI’s capabilities with human wisdom, ethics, and expertise. Are we up to the task? Only time and collective action will tell.
Related Articles
- AI in Telehealth: Revolutionizing Remote Care
- Navigating the Next: AI’s Role in Healthcare Predictive Analytics
- Transforming Medical Imaging with AI Capabilities in Radiology
- AI in Genetic Data Analysis: Personalizing Medicine
- AI in Diagnostics: A New Era of Precision and Accuracy
- Smart Hospitals: The Role of AI in hospital operations
- Mental Health Tech: AI-powered Mental Health Apps for Assessment and Therapy
- AI-Powered Drug Discovery: A Revolution in Pharma
- Medical Robots Enhanced by AI: Transforming Surgeries and Care
- Wearable Health Tech: AI-driven health wearables
- AI’s role in chronic disease management
- Neural Interfaces and AI: Bridging Minds and Machines
- Digital Health Platforms: The Power of AI Integration
- AI in Epidemiology: Predicting and Controlling Outbreaks
- AI in Rehabilitation: Personalized Recovery Pathways
- AI in Predictive and Preventive Care: A Proactive Approach
- AI in Home Healthcare: Personal Health Management
- Revolutionise Healthcare with IPA Tools: Streamlining Processes, Improving Efficiency, and Enhancing Patient Care
- Other Articles on AI Usage in Healthcare
- Other Articles on AI usage in different industries
Forbes Articles related to AI usage in Healthcare: