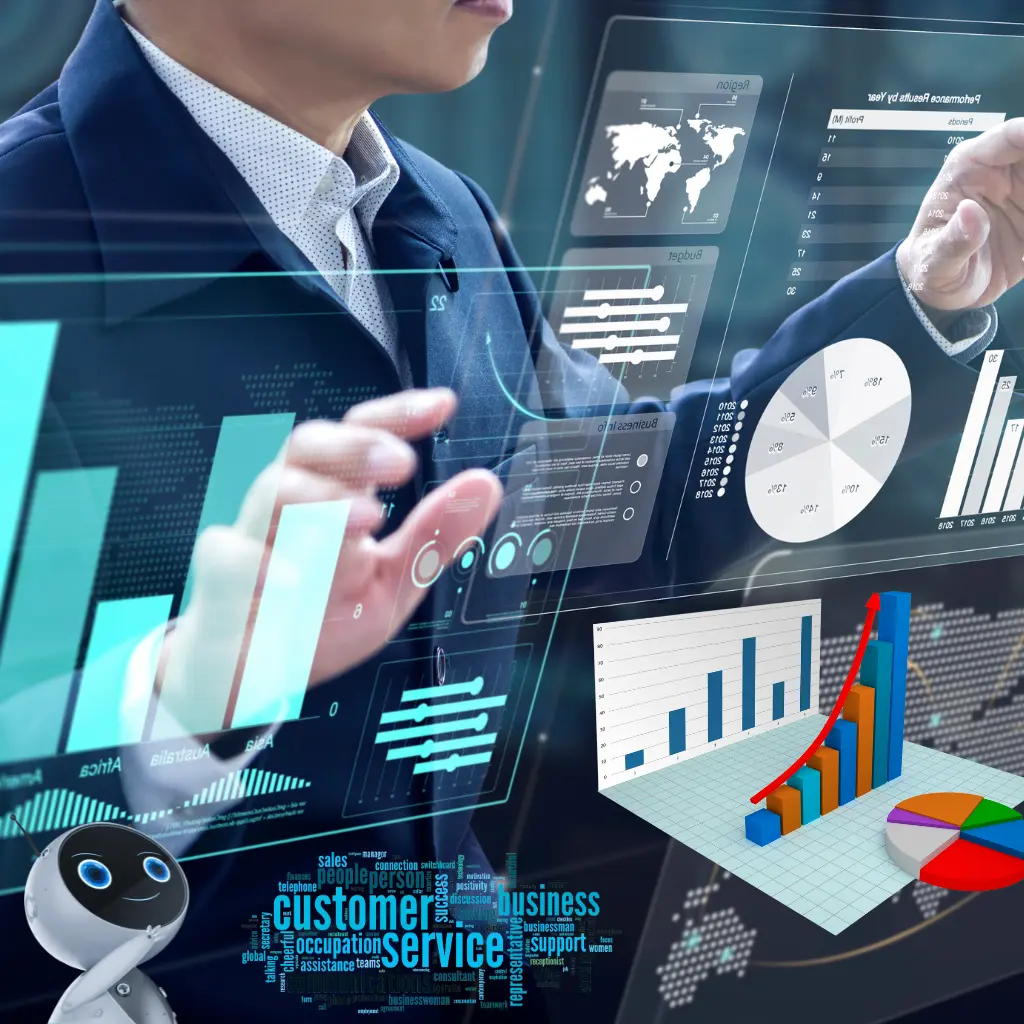
Revolutionizing Customer Experience
Unleashing Advanced Tools for Feedback and Survey Analysis!
As our company embarks on a transformative journey within the customer service domain, the focus on leveraging cutting-edge technology is paramount. In our 15 years of industry presence, we recognize the need to streamline and revolutionize our processes through automation and integrating new AI technologies.
The critical directive in this pursuit is the implementation of Customer Service Tools for Customer Feedback and Survey Analysis. This discussion centres on the strategic deployment of advanced tools, including sentiment analysis, survey response interpretation, trend analysis, satisfaction prediction, and NLP-based feedback summarization.
By delving into the requisite business and software knowledge, hardware infrastructure, training protocols, necessary integrations, and comparative market analysis, we aim to chart a course that propels us as a market leader and elevates the overall customer experience to new heights.
Table of Contents
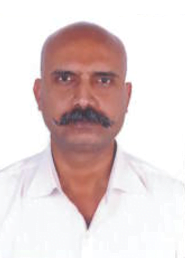
Arindam Roy
An Automation Consultant with 25+ years of IT Experience
5 Customer Feedback and Survey Analysis Tool ideas for the Customer Service
Customer Service Tools for Customer Feedback and Survey Analysis:
1. Sentiment Analysis Tool:
This tool employs Natural Language Processing (NLP) and machine learning (ML) algorithms to analyze and categorize sentiments expressed in customer feedback. By identifying and classifying positive, negative, or neutral sentiments, the Sentiment Analysis Tool provides a comprehensive understanding of customer emotions, allowing companies to address issues proactively and enhance customer satisfaction.
2. Automated Survey Response Analysis:
The Automated Survey Response Analysis tool utilizes advanced AI techniques to analyze and interpret customer survey responses automatically. By extracting meaningful insights from survey data, this tool streamlines the analysis process, enabling companies to identify patterns, preferences, and areas for improvement quickly. It enhances efficiency in handling large volumes of survey responses, saving time and resources while ensuring a more accurate analysis.
3. NLP-Based Feedback Summarization:
Leveraging Natural Language Processing, the NLP-Based Feedback Summarization tool focuses on summarizing and extracting crucial insights from lengthy customer feedback. This tool condenses extensive comments into concise summaries, making it easier for customer service teams and decision-makers to grasp key points quickly. This streamlines the feedback review process, facilitating faster response times and targeted action plans.
4. Customer Satisfaction Prediction Tool:
The Customer Satisfaction Prediction Tool applies machine learning algorithms to predict customer satisfaction based on feedback and interactions. This tool provides actionable predictions by correlating historical data with satisfaction outcomes. Companies can use these predictions to preemptively address potential issues, prioritize customer engagement efforts, and ultimately enhance overall customer satisfaction.
5. Trend Analysis in Customer Feedback:
The Trend Analysis in Customer Feedback tool utilizes AI to identify and analyze trends within customer feedback. By examining patterns and themes in feedback data, this tool aids in strategic decision-making. It enables companies to avoid issues, capitalize on positive trends, and align their customer service strategies with evolving customer preferences. The insights gained from trend analysis contribute to more informed business decisions and continuous improvement in customer service.
In summary, these Customer Service Tools for Customer Feedback and Survey Analysis leverage cutting-edge AI technologies to streamline and enhance various aspects of the customer feedback process. From sentiment analysis to trend identification, these tools empower companies to proactively address customer needs, optimize tasks, and maintain their position as market leaders in customer service.
Sentiment analysis of customer feedback
Business Knowledge Required:
- Customer Feedback Analysis: An in-depth understanding of customer service dynamics, including joint pain points and satisfaction areas, is required to accurately interpret and contextualize sentiments expressed in feedback.
- Industry-Specific Nuances: Familiarity with industry-specific nuances that impact customer sentiments ensures that the sentiment analysis models are tailored to the business’s unique characteristics.
- Legal and Ethical Considerations: Knowledge of data privacy and ethical considerations for feedback analysis, compliance with regulations, and maintenance of customer trust.
Software Knowledge Needed:
- NLP and Machine Learning: Proficiency in natural language processing (NLP) and machine learning (ML) algorithms to develop models capable of accurately analyzing and categorizing sentiments expressed in customer feedback.
- Data Processing and Storage: Skills in data processing and storage technologies to securely handle and store large volumes of customer feedback data.
- User Interface Design: Understanding user interface design to create user-friendly dashboards to visualize sentiment analysis results and extract actionable insights.
Hardware Needed to Run the Tools:
- Computational Resources: Adequate computational resources, potentially utilizing cloud services, to handle the processing demands of NLP and machine learning algorithms for sentiment analysis.
- Storage Infrastructure: Robust storage infrastructure to securely store and manage the vast customer feedback data generated over time.
Training Required to Run the Tools:
- Tool Operation Training: This training is for customer service teams to use the sentiment analysis tool, interpret results, and incorporate insights into their daily operations.
- Continuous Model Improvement: Training for data scientists or analysts responsible for continuously refining and improving the sentiment analysis models based on evolving language patterns and customer expressions.
Integrations Necessary:
- Feedback Collection Systems: Integration with existing feedback collection systems to seamlessly gather and feed customer feedback data into the sentiment analysis tool.
- Customer Relationship Management (CRM) Tools: Integration with CRM tools to link sentiment analysis results with individual customer profiles, providing a holistic view of customer sentiment over time.
Comparative Tools Already Available in the Market:
- IBM Watson Natural Language Understanding: Offers advanced sentiment analysis capabilities with additional features like emotion analysis and entity recognition.
- Google Cloud Natural Language API: A powerful tool for sentiment analysis, providing accurate and context-aware insights from customer feedback.
Recommendation:
Considering the specialized nature of sentiment analysis tools and the availability of robust solutions in the market, it is recommended that a company buy and customize an existing tool. This approach allows the company to leverage the advancements in NLP and machine learning offered by established providers while tailoring the tool to align with specific industry and business requirements within Customer Service Tools for Customer Feedback and Survey Analysis.
Cost/Benefits Analysis on the Recommendation:
- Costs:
- Licensing Fees: Incurred costs for purchasing a subscription or licensing the chosen sentiment analysis tool.
- Customization Costs: Initial development costs for customizing the tool to align with industry-specific nuances and business requirements.
- Benefits:
- Time-to-implementation: Implementation is faster than building from scratch, allowing the company to quickly benefit from advanced sentiment analysis capabilities.
- Accuracy and Reliability: Access to the expertise of established sentiment analysis tool providers, ensuring high accuracy and reliability in analyzing customer sentiments.
- Scalability: The scalability of existing tools allows the organization to adapt and expand sentiment analysis efforts based on evolving customer service needs.
In conclusion, the recommendation to buy and customize an existing sentiment analysis tool balances efficiency, accuracy, and customization within Customer Service Tools for Customer Feedback and Survey Analysis.
Automated survey response analysis
Business Knowledge Required:
- Survey Design and Structure: Understanding effective survey design and structure ensures the automated analysis tool can interpret responses accurately and in the context of the survey questions.
- Domain-Specific Knowledge: To interpret survey responses accurately within the relevant context, one must be familiar with industry-specific terminology, jargon, and nuances.
- Customer Behavior Understanding: In-depth knowledge of customer behaviour and preferences to interpret responses that reflect customer segments’ varying expectations and sentiments.
Software Knowledge Needed:
- Natural Language Processing (NLP): Proficiency in NLP to understand and process the natural language used in survey responses, extracting meaningful insights.
- Machine Learning Algorithms: Effective categorization and analysis of survey responses can be achieved through knowledge of machine learning algorithms for classification and clustering.
- Data Processing and Analytics: Skills in data processing and analytics tools to manage large volumes of survey data, derive actionable insights, and generate reports.
Hardware Needed to Run the Tools:
- Computational Resources: Adequate computing power, potentially leveraging cloud services, to handle the processing demands of NLP and machine learning algorithms for survey response analysis.
- Storage Infrastructure: Robust storage infrastructure to securely store and manage the vast survey response data generated over time.
Training Required to Run the Tools:
- Tool Operation Training: Customer service teams will be trained to use the automated survey response analysis tool, interpret results, and apply insights to improve customer service processes.
- Continuous Model Improvement: Training for data scientists or analysts who continuously refine and improve analysis models based on evolving language patterns and customer feedback.
Integrations Necessary:
- Survey Platforms: Integration with survey platforms to seamlessly collect and import survey response data into the analysis tool.
- Customer Relationship Management (CRM) Systems: Link survey responses with CRM tools for better customer insights.
Comparative Tools Already Available in the Market:
- Qualtrics: This product provides features to analyze survey responses, such as sentiment analysis and categorization of reactions.
- SurveyMonkey: Provides essential analysis tools for survey responses, including data visualization and trend analysis.
Recommendation:
Considering the specialized nature of survey response analysis tools and the availability of existing solutions in the market, it is recommended that a company buy and customize an existing tool. This approach allows the company to leverage the advancements in NLP and machine learning offered by established providers while tailoring the tool to align with specific industry and business requirements within Customer Service Tools for Customer Feedback and Survey Analysis.
Cost/Benefits Analysis on the Recommendation:
- Costs:
- Licensing Fees: Incurred costs for purchasing a subscription or licensing the chosen survey response analysis tool.
- Customization Costs: Initial development costs for customizing the tool to align with industry-specific nuances and business requirements.
- Benefits:
- Time-to-Implementation: Implementation is faster than building from scratch, allowing the company to benefit from advanced survey response analysis capabilities quickly.
- Accuracy and Reliability: Access to the expertise of established tool providers ensures high accuracy and reliability in analyzing survey responses.
- Scalability: The scalability of existing tools allows the organization to adapt and expand survey response analysis efforts based on evolving customer service needs.
In conclusion, the recommendation to buy and customize an existing survey response analysis tool balances efficiency, accuracy, and customization within Customer Service Tools for Customer Feedback and Survey Analysis.
NLP-based feedback summarization
Business Knowledge Required:
- Customer Service Domain Expertise: A profound understanding of the customer service domain, including industry-specific jargon, common customer pain points, and nuances in customer feedback, is essential. This knowledge helps develop algorithms that can accurately extract critical insights and sentiments from customer comments.
- Feedback Analysis Best Practices: Familiarity with best practices in feedback analysis is crucial for designing algorithms that prioritize and summarize the most relevant information from lengthy customer feedback. Knowledge of the business context ensures that the summaries provide actionable insights.
- Customer Behavior Understanding: In-depth knowledge of customer behaviour and preferences is necessary to interpret feedback accurately and capture customer segments’ varying expectations and sentiments.
Software Knowledge Needed:
- Natural Language Processing (NLP): Proficiency in NLP techniques is crucial for developing algorithms capable of understanding natural language’s structure, context, and semantics in customer feedback. This includes sentiment analysis, entity recognition, and topic modelling.
- Machine Learning Algorithms: Machine learning algorithms, especially text summarisation-related ones, are crucial for extracting critical insights from lengthy customer feedback.
- Data Processing and Analysis: Skills in data processing and analysis tools are necessary to handle large volumes of customer feedback data efficiently. This includes preprocessing, cleaning, and structuring the data for effective NLP-based summarization.
Hardware Needed to Run the Tools:
- Computational Resources: Adequate computing power is required, and utilizing cloud services may be beneficial to handle the processing demands of NLP algorithms for feedback summarization.
- Storage Infrastructure: Robust storage infrastructure is needed to securely store and manage the vast amount of customer feedback data and the generated summaries.
Training Required to Run the Tools:
- Tool Operation Training: Customer service teams will be trained to use the NLP-based feedback summarization tool, interpret summarized insights, and incorporate them into decision-making processes.
- Continuous Model Improvement: Training for data scientists or analysts who continuously refine and improve summarization models based on evolving language patterns and customer feedback.
Integrations Necessary:
- Feedback Collection Platforms: Integration with feedback collection platforms to seamlessly gather and import customer feedback data into the summarization tool.
- Customer Relationship Management (CRM) Systems: CRM tools can be integrated to link feedback insights with customer profiles for a complete view of customer sentiments.
Comparative Tools Already Available in the Market:
- MonkeyLearn: Offers NLP-based text analysis tools, including sentiment analysis and summarization, suitable for customer feedback analysis.
- TextRazor: NLP APIs extract entities and sentiments and summarize insights from the text that apply to customer feedback data.
Recommendation:
Given the specialized nature of NLP-based feedback summarization and the availability of existing solutions, it is recommended that a company buy and customize an existing tool. This approach allows the company to benefit from established providers’ expertise in NLP while tailoring the tool to align with specific industry and business requirements within Customer Service Tools for Customer Feedback and Survey Analysis.
Cost/Benefits Analysis on the Recommendation:
- Costs:
- Licensing Fees: Incurred costs for purchasing a subscription or licensing the chosen feedback summarization tool.
- Customization Costs: Initial development costs for customizing the tool to align with industry-specific nuances and business requirements.
- Benefits:
- Time-to-Implementation: Implementation is faster than building from scratch, allowing the company to benefit quickly from advanced feedback summarization capabilities.
- Accuracy and Reliability: Access to the expertise of established tool providers ensures high accuracy and reliability in summarizing customer feedback.
- Scalability: The scalability of existing tools allows the organization to adapt and expand feedback summarization efforts based on evolving customer service needs.
In conclusion, purchasing and customizing an existing feedback summarization tool is recommended. This balances efficiency, accuracy, and customization in Customer Service Tools for Customer Feedback and Survey Analysis.
Customer satisfaction prediction tool
Business Knowledge Required:
- Understanding of Customer Service Metrics: A deep understanding of key customer service metrics, including customer satisfaction indicators, is essential. This knowledge forms the foundation for identifying relevant features and patterns in feedback and interactions.
- Domain-specific Expertise: Industry knowledge is required to ensure that the machine learning model captures nuances unique to the business domain, aligning the predictions with the specific factors influencing customer satisfaction in that industry.
- Feedback Analysis Best Practices: Familiarity with best practices in feedback analysis to interpret and preprocess customer feedback data effectively for input into the machine learning model.
Software Knowledge Needed:
- Machine Learning Algorithms: Proficiency in machine learning algorithms, particularly those related to predictive analytics, regression, and classification, is crucial for building a robust model that accurately predicts customer satisfaction levels.
- Data Processing and Analysis: Skills in data processing and analysis tools are necessary to clean, preprocess, and structure considerable customer feedback and interaction data datasets for training the machine learning model.
- Programming Languages: To implement and deploy the prediction tool, you must be proficient in programming languages such as Python or R, commonly used in machine learning development.
Hardware Needed to Run the Tools:
- Computational Resources: Adequate computing power, potentially leveraging cloud services, to handle the computational demands of training and running machine learning models for customer satisfaction prediction.
- Storage Infrastructure: Robust storage infrastructure to securely store and manage historical customer feedback and interaction data, enabling continuous model training and improvement.
Training Required to Run the Tools:
- Tool Operation Training: Training for customer service teams on utilizing the customer satisfaction prediction tool, interpreting predictions, and integrating insights into customer service processes.
- Model Monitoring and Improvement: Training for data scientists or analysts responsible for monitoring the model’s performance, retraining it as needed, and continuously improving its accuracy over time.
Integrations Necessary:
- Customer Relationship Management (CRM) Tools: Integration with CRM systems to access and analyze customer interaction data, ensuring a comprehensive input for the prediction model.
- Feedback Collection Platforms: Integration with feedback collection platforms to incorporate customer sentiment data into the prediction model.
Comparative Tools Already Available in the Market:
- Zendesk Satisfaction Prediction: A predictive analytics tool specifically designed for customer satisfaction prediction, leveraging machine learning algorithms.
- Salesforce Einstein Analytics: Provides predictive analytics features, including tools for predicting customer satisfaction based on historical data.
Recommendation:
Given predictive analytics’ complexity and the availability of existing tools in the market, it is recommended that a company buy and customize an off-the-shelf predictive analytics tool. This approach allows the company to benefit from the expertise of established providers while tailoring the tool to align with specific industry and business requirements within the context of Customer Service Tools for Customer Feedback and Survey Analysis.
Cost/Benefits Analysis on the Recommendation:
- Costs:
- Licensing Fees: Incurred costs for purchasing a subscription or licensing the chosen predictive analytics tool.
- Customization Costs: Initial development costs for customizing the tool to align with industry-specific nuances and business requirements.
- Benefits:
- Time-to-Implementation: Faster implementation compared to building from scratch, allowing the company to benefit from advanced predictive analytics capabilities quickly.
- Accuracy and Reliability: Access to the expertise of established predictive analytics tool providers ensures high accuracy and reliability in predicting customer satisfaction levels.
- Scalability: The scalability of existing tools allows the organization to adapt and expand predictive analytics efforts based on evolving customer service needs.
In conclusion, the recommendation to buy and customize an existing predictive analytics tool balances efficiency, accuracy, and customization within Customer Service Tools for Customer Feedback and Survey Analysis.
Trend analysis in customer feedback
Business Knowledge Required:
- Customer Service Metrics Understanding: Deep understanding of customer service metrics and KPIs to identify relevant trends in customer feedback. This includes knowledge of key performance indicators such as customer satisfaction scores, Net Promoter Score (NPS), and customer churn rates.
- Industry Trends Awareness: Awareness of industry-specific trends and market dynamics to contextualize the trends identified in customer feedback. Understanding how broader industry shifts impact customer sentiment is crucial for strategic decision-making.
- Data Interpretation Skills: Strong data interpretation skills to analyze trends in customer feedback accurately and derive actionable insights. This involves identifying patterns, correlations, and anomalies in the data that may impact business strategies.
Software Knowledge Needed:
- Data Analysis Tools: Proficiency in data analysis tools such as Python’s pandas library, R, or SQL for processing and analyzing large volumes of customer feedback data.
- Machine Learning Algorithms: Knowledge of machine learning (ML) algorithms, particularly those related to trend detection and time-series analysis, to develop models capable of identifying and forecasting trends in customer feedback.
- Data Visualization Tools: Familiarity with tools such as Tableau or Power BI is necessary to create visually appealing and informative dashboards for presenting trend analysis results to stakeholders.
Hardware Needed to Run the Tools:
- Computational Resources: Adequate computing power, potentially leveraging cloud services, to handle the processing demands of analyzing large datasets and running machine learning algorithms for trend analysis.
- Storage Infrastructure: Robust storage infrastructure to securely store and manage historical customer feedback data and trend analysis results.
Training Required to Run the Tools:
- Tool Operation Training: Training for relevant teams on how to use the trend analysis tool, interpret trend analysis results, and integrate insights into strategic decision-making processes.
- Continuous Learning: Ongoing training for data analysts or data scientists responsible for maintaining and updating the trend analysis models to adapt to evolving customer feedback patterns.
Integrations Necessary:
- Customer Relationship Management (CRM) Tools: Integration with CRM systems to access and analyze customer feedback data collected through various channels, including emails, surveys, and social media.
- Feedback Collection Platforms: Integration with feedback collection platforms to gather real-time customer feedback data and incorporate it into the trend analysis tool for continuous monitoring.
Comparative Tools Already Available in the Market:
- Medallia Experience Cloud: Offers advanced analytics capabilities for trend analysis in customer feedback, including sentiment analysis, root cause analysis, and predictive analytics.
- Qualtrics XM: Provides tools for tracking and analyzing trends in customer feedback across multiple touchpoints, enabling organizations to identify patterns and take proactive measures to improve customer experience.
Recommendation:
Given the complexity of trend analysis in customer feedback and the availability of existing tools in the market, it is recommended that a company buy and customize an off-the-shelf analytics platform. This approach allows the company to benefit from established providers’ expertise while tailoring the tool to align with specific industry and business requirements within Customer Service Tools for Customer Feedback and Survey Analysis.
Cost/Benefits Analysis on the Recommendation:
- Costs:
- Licensing Fees: Incurred costs for purchasing a subscription or licensing the chosen analytics platform.
- Customization Costs: Initial development costs for customizing the tool to align with industry-specific nuances and business requirements.
- Benefits:
- Time-to-Implementation: Implementation is faster than building from scratch, allowing the company to benefit from advanced trend analysis capabilities quickly.
- Accuracy and Reliability: Access to the expertise of established analytics platform providers ensures high accuracy and reliability in identifying trends in customer feedback.
- Scalability: The scalability of existing tools allows the organization to adapt and expand trend analysis efforts based on evolving customer service needs.
In conclusion, the recommendation to buy and customize an existing trend analysis tool balances efficiency, accuracy, and customization within Customer Service Tools for Customer Feedback and Survey Analysis.
Conclusion
In conclusion, integrating advanced Customer Service Tools for Customer Feedback and Survey Analysis is a pivotal strategy for our company’s continued success in the evolving customer service landscape. As a seasoned player in the industry for 15 years, our commitment to automating processes and harnessing new AI technologies positions us as a market leader. The discussed tools, ranging from sentiment analysis and survey response interpretation to trend analysis and satisfaction prediction, underscore our dedication to enhancing customer experience through cutting-edge solutions.
The multifaceted business knowledge required, encompassing domain expertise, feedback analysis best practices, and a nuanced understanding of customer behaviour, forms the bedrock for developing these tools. Complemented by a comprehensive software knowledge base in NLP, machine learning (ML), and data analytics, these tools promise to revolutionize our approach to customer feedback and survey analysis.
The hardware infrastructure, including computational resources and storage capabilities, aligns with the robustness required to handle the sophisticated algorithms and vast datasets involved. Training programs ensure that our teams are well-equipped to operate, maintain, and continuously improve these tools, fostering a culture of adaptability and innovation.
By considering integrations with CRM systems, feedback collection platforms, and other essential channels, we ensure a seamless data flow into our tools, providing a holistic view of customer sentiments. Comparative analysis of existing market tools informs our decision-making, ultimately leading to buying and customizing off-the-shelf solutions, balancing efficiency, accuracy, and customization.
The cost/benefits analysis emphasizes the advantages of swift implementation, access to expertise, and scalability, solidifying our choice to embrace Customer Service Tools for Customer Feedback and Survey Analysis. This strategic adoption positions our company at the forefront of the customer service industry, ensuring we meet and exceed customer expectations in an ever-evolving marketplace.
Related Articles
- 50 AI Tools for the Customer Services Domain
- 5 Facial Recognition and Emotion Analysis Tools of Customer Service
- 5 Customer Service Speech Recognition and Processing Tools
- 5 Customer Service Predictive Analytics and Recommendation
- 5 Customer Service Tolls for Automation and RPA
- 5 Customer Service Natural Language Processing (NLP) Tools
- 5 Customer Service Tools for Knowledge Management
- 5 Customer Service Tools for Continuous Learning and Improvement
- 5 Customer Service Tools for Social Media Monitoring
- 5 Customer Service Tools for Data Security and Privacy