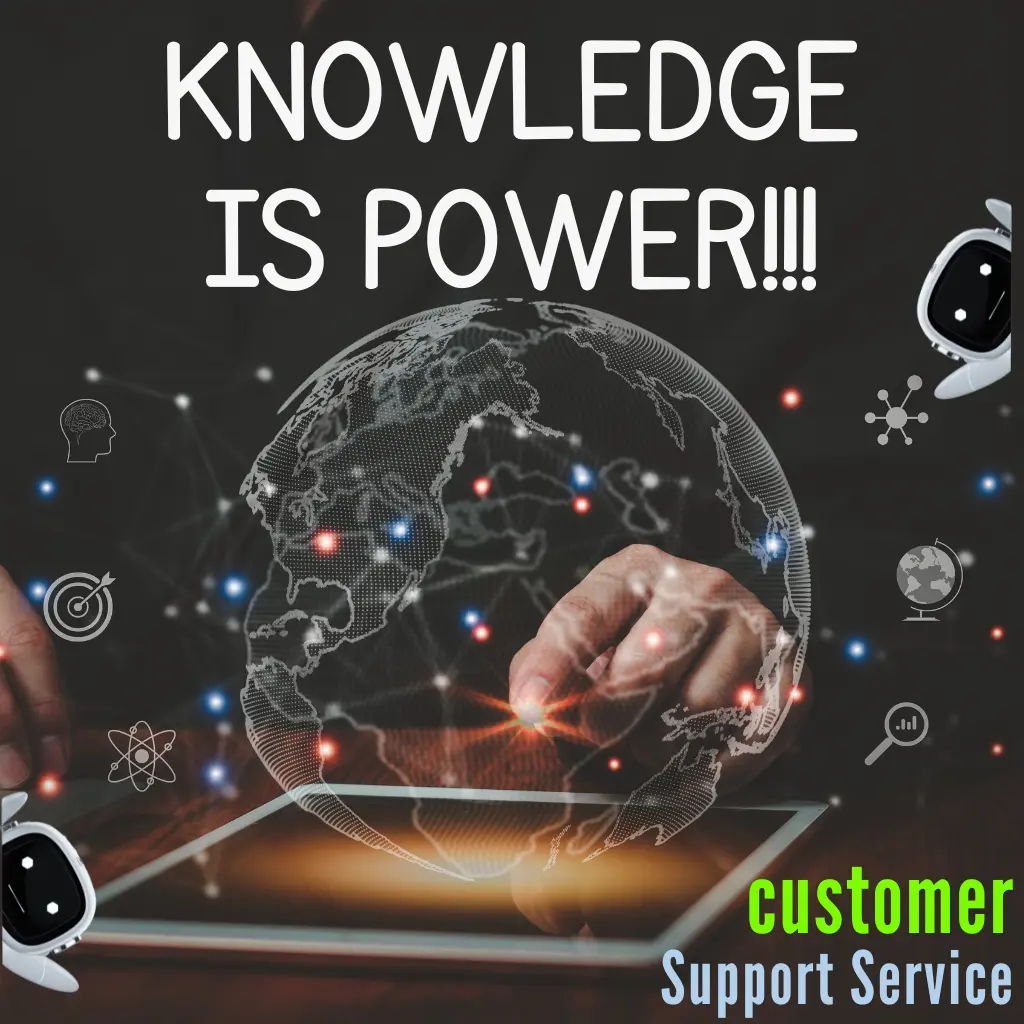
Revolutionizing Customer Service
Unleashing the Power of Knowledge Management Systems
Integrating advanced technologies has become paramount for staying competitive and enhancing operational efficiency in the dynamic customer service landscape. As a market leader in the Customer Service domain for 15 years, the strategic imperative is to automate processes and harness the power of new AI technologies.
This discussion centres on the pivotal development of Customer Service Tools for Knowledge Management, a comprehensive suite that aims to revolutionize information organization, retrieval, and continuous improvement.
These tools, which span content categorization, knowledge extraction, automated content updates, and intelligent search, are designed to empower organizations to manage and leverage their vast reservoirs of information efficiently.
This discussion explains the essential factors to consider when adopting and customizing business software tools to improve customer service. It covers hardware requirements, training needs, software knowledge, integrations, and market tools. The focus is on elevating customer service excellence. We’ll use simple, everyday language to make it easy to understand. We’ll also keep sentences short and direct and organize the information logically.
Table of Contents
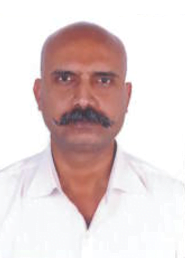
Arindam Roy
An Automation Consultant with 25+ years of IT Experience
5 Knowledge Management Tool ideas for the Customer Service
Customer Service Tools for Knowledge Management:
1. AI-driven knowledge base for self-service:
This tool employs advanced AI algorithms to organize and continually update a comprehensive knowledge base. By leveraging machine learning, it intelligently categorizes and structures information, making it easily accessible to customers. This self-service solution empowers users to find relevant information independently, enhancing their overall experience and reducing the need for direct customer support.
2. Content categorization and tagging tool:
The content categorization and tagging tool utilizes machine learning techniques to categorize and tag diverse content within the knowledge base automatically. This intelligent system enhances information organization, making it more accessible and user-friendly. By efficiently categorizing content, it streamlines the customer’s search experience and facilitates the ongoing maintenance and growth of the knowledge base.
3. Knowledge extraction from unstructured data:
This tool leverages natural language processing (NLP) and machine learning to extract valuable insights from unstructured data sources. It efficiently transforms unstructured information into structured knowledge, contributing to a more robust and comprehensive knowledge base. By integrating this tool, the company can tap into a broader range of data, ensuring the knowledge base remains up-to-date and relevant to evolving customer needs.
4. Automated content updates based on user feedback:
This system incorporates AI to analyze user feedback effectively. By leveraging sentiment analysis and other machine learning techniques, the tool interprets customer input and identifies areas for improvement in the knowledge base. Automation ensures swift and accurate updates, reflecting customer preferences and addressing common concerns. This tool promotes a dynamic and responsive knowledge base, maintaining its relevance over time.
5. Intelligent search and retrieval system:
The AI-powered search and retrieval system uses advanced algorithms to improve the accuracy and efficiency of information retrieval. It goes beyond traditional keyword-based searches by utilizing machine learning to understand customer intent and context, resulting in precise and relevant search results that enhance the user experience and quickly direct customers to necessary information within the knowledge base.
In summary, these Customer Service Tools for Knowledge Management collectively form a sophisticated ecosystem that leverages AI technologies to optimize self-service, content organization, data extraction, user feedback analysis, and information retrieval within the customer service domain. Integrating these tools ensures a cutting-edge and efficient knowledge management system, reinforcing the company’s position as a market leader in customer service.
AI-driven knowledge base for self-service
Business Knowledge Required:
- Customer Service Processes: Understanding the end-to-end customer service processes, including information flow, common customer queries, and the overall customer journey.
- Content Management: Expertise in organizing and categorizing content, understanding user preferences, and ensuring the knowledge base aligns with customer needs.
- AI and NLP Concepts: Familiarity with AI and natural language processing (NLP) concepts to grasp the technical aspects of implementing AI-driven tools.
Software Knowledge Needed:
- AI Development: Proficiency in AI development tools and frameworks to create and deploy algorithms for the AI-driven knowledge base.
- Content Management Systems (CMS): Knowledge of CMS platforms to design and implement a user-friendly and easily updatable knowledge base.
- NLP Programming: Skills in programming languages used for NLP, such as Python or Java, to enable knowledge extraction from unstructured data.
Hardware Needed to Run the Tools:
- Sufficient Computing Resources: Adequate computing power, potentially utilizing cloud services, to handle the computational requirements of AI algorithms and content management.
- Data Storage Infrastructure: Robust data storage to accommodate the growing knowledge base and user data, ensuring quick retrieval.
Training Required to Run the Tools:
- AI and NLP Training: This training allows employees to manage and update the AI-driven knowledge base to understand AI and NLP concepts.
- Content Management System Training: Instruction for users responsible for maintaining and organizing content within the CMS knowledge base.
Integrations Necessary:
- User Feedback Systems: Integration with user feedback systems to enable automated content updates based on customer input.
- Search Engine Integration: Integration with search engines to enhance the intelligent search and retrieval system.
Comparative Tools Already Available in the Market:
- Zendesk Guide: A comprehensive knowledge management solution offering AI-driven features for self-service and content organization.
- Freshdesk: Known for its AI capabilities, Freshdesk provides tools for intelligent search and content categorization.
Recommendation:
Considering the availability of well-established tools in the market, it is recommended that the company explore, potentially buy, and customize an existing knowledge management platform. This approach allows the company to benefit from proven solutions while tailoring the tools to specific customer service processes and preferences.
Cost/Benefits Analysis on the Recommendation:
- Costs:
- Licensing Fees: Incurred costs for purchasing licenses or subscriptions for the chosen knowledge management platform.
- Customization Costs: Initial development costs for customizing the platform to align with specific customer service processes.
- Benefits:
- Time-to-Market: Faster implementation compared to building from scratch, leading to quicker availability of advanced knowledge management capabilities.
- Vendor Support: Access to vendor support and updates, ensuring the reliability and security of the knowledge management tools.
- Scalability: The scalability of existing platforms allows the organization to adapt and expand as customer service needs evolve.
In conclusion, the recommendation to buy and customize an existing knowledge management platform offers a balance between efficiency, reliability, and customization within the context of Customer Service Tools for Knowledge Management.
Content categorization and tagging tool
Business Knowledge Required:
- Content Management Processes: A deep understanding of the company’s content management processes, including how information is created, updated, and archived within the knowledge base.
- User Behavior Analysis: Insight into user behaviour and preferences to ensure that the categorization and tagging align with how users typically search for and interact with information.
- Industry-Specific Knowledge: Knowledge of industry-specific terminology and context to improve the accuracy of content categorization and tagging.
Software Knowledge Needed:
- Machine Learning and Data Science: Proficiency in machine learning algorithms and data science techniques to develop models for automatic content categorization and tagging.
- Programming Languages: Skills in programming languages such as Python or Java to implement and integrate machine learning algorithms into the existing knowledge management system.
- Database Management: Knowledge of database management systems to efficiently store and retrieve tagged content, ensuring optimal performance.
Hardware Needed to Run the Tools:
- Sufficient Computing Resources: Adequate computing power, potentially using cloud services, to handle the computational demands of training and running machine learning models.
- Data Storage Infrastructure: Robust data storage infrastructure to manage the increasing volume of categorized and tagged content.
Training Required to Run These Tools Once Created:
- Content Moderators: Training for content moderators or administrators responsible for overseeing and refining the categorization and tagging processes.
- System Administrators: Instruction for system administrators to ensure the seamless integration and operation of the tool within the overall knowledge management system.
Integrations Necessary:
- Content Management System (CMS): Integration with the existing CMS to automatically apply tags and categories to new and existing content.
- Search Engine Integration: Collaboration with search engines to improve search results by utilizing the categorized and tagged content.
Comparative Tools Already Available in the Market:
- MonkeyLearn: An AI-based platform that offers text analysis and categorization tools, including pre-built models for content classification.
- Amazon Comprehend: A cloud-based natural language processing service that provides sentiment analysis and entity recognition, which can be utilized for content categorization.
Recommendation:
Given the complexity of machine learning and the availability of specialized tools in the market, it is recommended that you explore and buy a pre-built content categorization and tagging tool. Customization may be required to align it with specific business needs.
Cost/Benefits Analysis on the Recommendation:
- Costs:
- Licensing Fees: Incurred costs for purchasing licenses or subscriptions for the chosen content categorization tool.
- Customization Costs: Potential expenses for customizing the tool to fit seamlessly with the existing knowledge management system.
- Benefits:
- Time-to-Implementation: Faster implementation compared to building from scratch, leading to quicker improvements in knowledge base organization.
- Advanced Features: Access to advanced features and continuous updates provided by specialized tools, enhancing the overall effectiveness of content categorization.
- Technical Support: Ongoing technical support from the tool provider, ensuring reliability and addressing any issues promptly.
In conclusion, the recommendation to buy and potentially customize an existing content categorization and tagging tool offers a pragmatic balance between efficiency, reliability, and customization within Customer Service Tools for Knowledge Management.
Knowledge extraction from unstructured data
Business Knowledge Required:
- Data Sources Understanding: Comprehensive knowledge of the various unstructured data sources within the customer service domain, such as customer communications, feedback, and other textual information.
- Relevance Criteria Definition: Expertise in defining the criteria for relevance in extracted information, aligning with customer service processes’ specific needs and objectives.
- Industry-Specific Context: Understanding industry-specific terminology and context to ensure accurate extraction and interpretation of information.
Software Knowledge Needed:
- Natural Language Processing (NLP): Proficiency in NLP techniques and frameworks to analyze and interpret human language within unstructured data.
- Machine Learning Algorithms: Skills in developing and implementing machine learning (ML) algorithms, including supervised and unsupervised learning, to train models and extract relevant information.
- Programming Languages: Proficiency in programming languages, i.e. Python or Java, for implementing NLP and machine learning algorithms.
Hardware Needed to Run the Tools:
- Computational Resources: Adequate computing power, potentially leveraging cloud services, to handle the computational demands of processing and analyzing unstructured data.
- Storage Infrastructure: Robust data storage infrastructure to store both the unstructured data and the extracted knowledge, ensuring accessibility and retrieval efficiency.
Training Required to Run These Tools Once Created:
- NLP Understanding: Training for users responsible for overseeing the knowledge extraction process to understand the principles and limitations of natural language processing.
- Data Validation and Correction: Instruction on validating and correcting extracted information to enhance accuracy and relevance.
Integrations Necessary:
- Data Sources Integration: Integration with various data sources, including customer service databases, emails, and feedback platforms, to streamline the extraction process.
- Knowledge Base Integration: Collaboration with the knowledge base system to ensure seamless incorporation of extracted information into the existing repository.
Comparative Tools Already Available in the Market:
- MonkeyLearn: A text analysis tool that offers capabilities for extracting insights from unstructured data, including sentiment analysis and entity recognition.
- Microsoft Azure Text Analytics: A cloud-based service providing NLP capabilities, including sentiment analysis, key phrase extraction, and language detection.
Recommendation:
Considering the complexity of NLP and machine learning and the availability of specialized tools in the market, it is recommended that you explore and buy an existing knowledge extraction tool. Customization may be required to align it with specific business requirements.
Cost/Benefits Analysis on the Recommendation:
- Costs:
- Licensing Fees: Incurred costs for purchasing licenses or subscriptions for the chosen knowledge extraction tool.
- Customization Costs: Potential expenses for customizing the tool to fit seamlessly with the existing knowledge management system.
- Benefits:
- Time-to-Implementation: Faster implementation compared to building from scratch, leading to quicker improvements in knowledge extraction from unstructured data.
- Advanced Features: Access to advanced features and continuous updates provided by specialized tools, enhancing the overall effectiveness of knowledge extraction.
- Technical Support: Ongoing technical support from the tool provider, ensuring reliability and addressing any issues promptly.
In conclusion, the recommendation to buy and customize an existing knowledge extraction tool offers a pragmatic balance between efficiency, reliability, and customization within the context of Customer Service Tools for Knowledge Management.
Automated content updates based on user feedback
Business Knowledge Required:
- Understanding of User Behavior: In-depth knowledge of customer behaviour and interaction patterns to interpret and analyze user feedback effectively.
- Content Relevance Criteria: Expertise in defining criteria for assessing the relevance and importance of user feedback to prioritize and implement updates.
- Industry-Specific Knowledge: Understanding industry-specific terminology and context ensures content updates align with customer needs and expectations.
Software Knowledge Needed:
- Natural Language Processing (NLP): Proficiency in NLP techniques to accurately analyze and understand user feedback, including sentiment analysis and entity recognition.
- Machine Learning Algorithms: Skills in implementing machine learning algorithms, such as recommendation systems, to predict and prioritize content updates based on user feedback.
- Programming Languages: Proficiency in programming languages, i.e. Python or Java, is required to develop algorithms and integrate them into the existing knowledge management system.
Hardware Needed to Run the Tools:
- Computational Resources: Adequate computing power, utilizing cloud services, to handle the computational demands of processing and analyzing user feedback in real-time.
- Storage Infrastructure: Robust data storage infrastructure to store and retrieve user feedback, historical data, and the corresponding knowledge base content.
Training Required to Run These Tools Once Created:
- User Feedback Analysts: Training for individuals responsible for interpreting and validating the results of the automated analysis to ensure accuracy and relevance.
- System Administrators: Instruction for system administrators to manage and monitor the automated content update system, including addressing any issues.
Integrations Necessary:
- User Feedback Platforms: Integration with existing user feedback platforms, such as customer support systems and survey tools, to gather and analyze feedback.
- Content Management System (CMS): Collaboration with the CMS to seamlessly implement content updates based on the insights derived from user feedback.
Comparative Tools Already Available in the Market:
- Medallia: A customer feedback management platform that provides AI-driven insights for improving customer experience.
- Clarabridge: Offers sentiment analysis and feedback management solutions, automating content updates based on user feedback.
Recommendation:
Considering the complexity of analyzing user feedback and the availability of specialized tools, it is recommended that you explore and buy an existing automated content update tool. Customization may be needed to align it with specific business requirements.
Cost/Benefits Analysis on the Recommendation:
- Costs:
- Licensing Fees: Incurred costs for purchasing licenses or subscriptions for the chosen automated content update tool.
- Customization Costs: Potential expenses for customizing the tool to fit seamlessly with the existing knowledge management system.
- Benefits:
- Time-to-Implementation: Implementing a solution quickly compared to building from scratch leads to quicker improvements in content updates based on user feedback.
- Advanced Features: Access to advanced features and continuous updates provided by specialized tools, enhancing the overall effectiveness of automated content updates.
- Technical Support: Ongoing technical support from the tool provider, ensuring reliability and addressing any issues promptly.
In conclusion, the recommendation to buy and customize an existing automated content update tool offers a pragmatic balance between efficiency, reliability, and customization within Customer Service Tools for Knowledge Management.
Intelligent search and retrieval system
Business Knowledge Required:
- Understanding of Customer Queries: In-depth knowledge of common customer queries and search patterns within the customer service domain to optimize search results.
- Content Relevance Criteria: Expertise in defining criteria for assessing the relevance and importance of retrieved information to meet customer expectations.
- Industry-Specific Context: Understanding industry-specific terminology and context to enhance the accuracy of search results.
Software Knowledge Needed:
- Natural Language Processing (NLP): Proficiency in NLP techniques to understand customer queries and match them with relevant content within the knowledge base.
- Machine Learning Algorithms: Skills in implementing machine learning algorithms, such as ranking algorithms, to prioritize search results based on relevance.
- Search Engine Technologies: Knowledge of search engine technologies to design and implement efficient search algorithms for quick and accurate information retrieval.
Hardware Needed to Run the Tools:
- Computational Resources: Adequate computing power, potentially utilizing cloud services, to handle the computational demands of processing search queries and ranking results.
- Storage Infrastructure: Robust data storage infrastructure to store and manage vast information within the knowledge base.
Training Required to Run These Tools Once Created:
- Search Analysts: Training for individuals responsible for monitoring and analyzing search patterns, identifying areas for improvement, and refining search algorithms accordingly.
- System Administrators: Instruction for system administrators to manage and monitor the intelligent search and retrieval system, ensuring optimal performance.
Integrations Necessary:
- Content Management System (CMS): Integration with the CMS to ensure real-time updates and synchronization of the knowledge base content with the search system.
- User Feedback Systems: Integration with user feedback systems to continuously improve search algorithms based on user interactions and preferences.
Comparative Tools Already Available in the Market:
- Elasticsearch: A widely-used open-source search and analytics engine known for its scalability and fast search capabilities.
- Algolia: A cloud-based search and discovery platform offering AI-driven search algorithms for enhanced relevance.
Recommendation:
Considering the complexity of search algorithms and the availability of specialized tools, exploring and buying an existing intelligent search and retrieval system is recommended. Customization may be required to align it with specific business requirements.
Cost/Benefits Analysis on the Recommendation:
- Costs:
- Licensing Fees: Incurred costs for purchasing licenses or subscriptions for the chosen intelligent search and retrieval tool.
- Customization Costs: Potential expenses for customizing the tool to fit seamlessly with the existing knowledge management system.
- Benefits:
- Time-to-Implementation: Faster implementation compared to building from scratch, leading to quicker improvements in search and retrieval capabilities.
- Advanced Features: Access to advanced features and continuous updates provided by specialized tools, enhancing the overall effectiveness of the intelligent search and retrieval system.
- Technical Support: Ongoing technical support from the tool provider, ensuring reliability and addressing any issues promptly.
In conclusion, the recommendation to buy and customize an existing intelligent search and retrieval system offers a pragmatic balance between efficiency, reliability, and customization within Customer Service Tools for Knowledge Management.
Conclusion
In conclusion, the imperative evolution of customer service towards automation and AI technologies underscores the significance of adopting advanced tools for effective Knowledge Management. The discussed suite of Customer Service Tools for Knowledge Management, encompassing content categorization, knowledge extraction, automated content updates, and intelligent search, reflects a strategic commitment to staying at the forefront of the industry after 15 years of experience. These tools demand a nuanced blend of business acumen and technical expertise, necessitating a deep understanding of customer behaviour, industry-specific nuances, and content management processes.
The software knowledge required spans natural language processing, machine learning, and proficiency in relevant programming languages. Adequate computational resources and robust data storage infrastructure are prerequisites for running these tools efficiently. The significance of training programs for user feedback analysts, system administrators, and content moderators must be balanced to ensure seamless operation and continuous improvement.
When considering integration points, connecting these tools with existing systems, such as content management platforms and user feedback systems, emerges as crucial. While comparative tools are available in the market, the recommendation leans towards a pragmatic approach to buying and customizing existing solutions. This approach balances the advantages of time-to-implementation, access to advanced features, and ongoing technical support against the associated costs. Embracing these Customer Service Tools for Knowledge Management is not merely a strategic choice but a pivotal step towards enhancing customer experience and operational efficiency and maintaining a pioneering position in the dynamic customer service landscape.
Related Articles
- 50 AI Tools for the Customer Services Domain
- 5 Facial Recognition and Emotion Analysis Tools of Customer Service
- 5 Customer Service Speech Recognition and Processing Tools
- 5 Customer Service Predictive Analytics and Recommendation
- 5 Customer Service Tolls for Automation and RPA
- 5 Customer Service Natural Language Processing (NLP) Tools
- 5 Customer Service Tools for Continuous Learning and Improvement
- 5 Customer Service Tools for Customer Survey Analysis
- 5 Customer Service Tools for Social Media Monitoring
- 5 Customer Service Tools for Data Security and Privacy