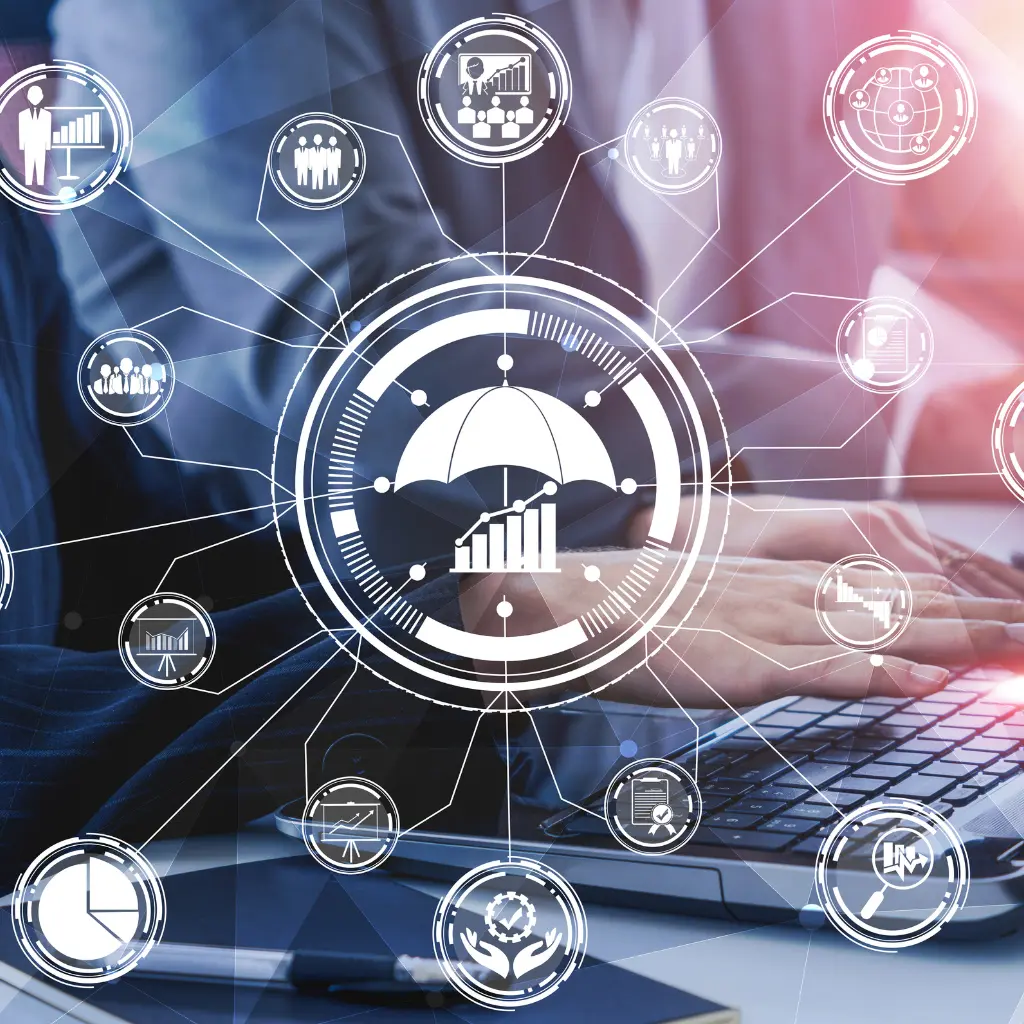
Fortifying Logistics
AI Tools for Enhanced Risk Management and Security in the Industry's Dynamic Landscape
In the dynamic landscape of the logistics industry, staying ahead of emerging risks is paramount. “AI Tools for Risk Management and Security in the Logistics Industry” represents a transformative approach to address the multifaceted challenges inherent in transportation and supply chain operations.
Integrating advanced artificial intelligence becomes imperative as the industry strives for efficiency, sustainability, and heightened security measures. From Sustainable Sourcing Guidance to Biometric Authentication for Access, each tool is meticulously designed to fortify logistics against potential threats.
This discussion delves into the intricacies of implementing these AI-driven solutions:
- Business knowledge prerequisites
- Software and hardware requirements
- Integration strategies
- Staff training needs
- Existing market tools
- Comprehensive cost-benefit analysis
Navigating logistics security is complex. This conversation will guide organizations to adopt intelligent risk management solutions that meet the unique demands of the logistics sector.
Table of Contents
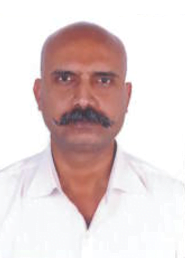
Arindam Roy
An Automation Consultant with 25+ years of IT Experience
5 AI Tool ideas for Risk Management and Security
AI-powered tools can enhance efficiency and mitigate risks in the Transportation and Logistics industry. Here’s an elaboration on the selected tools:
Cargo Security Monitoring:
- AI-driven Surveillance Systems: Implement advanced AI-powered video analytics to monitor cargo areas and warehouses. The system can automatically detect anomalies or suspicious activities, triggering alerts for further investigation.
- Predictive Analytics: Use machine learning to predict security threats from past data. Act now to safeguard your business. This can help in proactive decision-making and preventive measures.
Fraud Detection in Logistics:
- Pattern Recognition: Utilize machine learning algorithms to identify irregularities in transaction patterns, invoice data, or shipment routes that may indicate fraudulent activities.
- Anomaly Detection: Implement real-time monitoring systems to detect unusual behaviours, such as sudden changes in shipping routes, unexpected delays, or deviations from standard procedures.
Real-Time Incident Response:
- Automated Response Systems: Develop AI-driven systems that instantly respond to security incidents. This may involve automated communication with relevant stakeholders, shipment rerouting, or activating security protocols.
- Integration with IoT Devices: Connect AI systems with IoT devices and sensors to receive real-time data on the status of shipments and logistics operations, enabling immediate responses to incidents.
Biometric Authentication for Access:
- Facial Recognition: Implement biometric authentication for access control at critical points such as warehouses, loading docks, and transportation hubs.
- Fingerprint and Iris Scanning: Utilize fingerprints and iris scans to limit access to sensitive areas.
AI-Enhanced Cybersecurity:
- Predictive Cyber Threat Analysis: Use AI algorithms to analyze network traffic and identify potential cybersecurity threats. Taking a proactive approach can help prevent data breaches and system compromises.
- Behavioural Analysis: Implement AI-based systems that continuously monitor user and system behaviour, identifying unusual patterns that may indicate a cyber attack or unauthorized access.
Collectively, these AI tools contribute to a robust risk management and security framework in the transportation and logistics industry, providing real-time monitoring, proactive threat detection, and efficient incident response. Integrating these tools can also help create a more resilient and secure supply chain ecosystem.
Cargo Security Monitoring
Business Knowledge Requirements:
- Logistics and Security Expertise:
- Understanding of logistics operations, cargo handling, and the specific security challenges in the transportation industry.
- Familiarity with regulations and compliance standards related to cargo security.
- AI and Surveillance Technology:
- Knowledge of AI algorithms for video analytics and anomaly detection.
- Understanding of surveillance technology and its applications in monitoring cargo areas.
- Risk Management:
- Conducting practical risk assessment and management can help to identify potential threats and vulnerabilities in the supply chain.
- Knowledge of incident response protocols and crisis management in logistics.
Software Requirements:
- AI Algorithms for Video Analytics:
- Development of AI algorithms capable of analyzing video feeds in real-time to detect anomalies and security threats.
- Integration of machine learning models for continuous improvement in threat detection accuracy.
- Predictive Analytics Models:
- Implement machine learning models for predictive analytics to identify potential security threats based on historical data.
- Integration with existing risk assessment tools for a comprehensive security analysis.
- Alerting and Reporting System:
- Designing an alerting system to notify security personnel when suspicious activities are detected in real-time.
- Implementing reporting features to generate insights and support decision-making.
Hardware Requirements:
- High-Resolution Cameras:
- High-resolution cameras were deployed in cargo areas and warehouses to capture detailed images for analysis.
- Integration with existing surveillance infrastructure for optimal coverage.
- Powerful Processing Units:
- Adequate computing power to process and analyze video data in real time.
- Consideration of edge computing solutions for immediate on-site processing.
Integration Requirements to Existing Toolset:
- Security Information and Event Management (SIEM) Integration:
- Integration with SIEM systems to correlate security events with other relevant data for a holistic security analysis.
- Collaboration with existing security infrastructure to streamline incident response.
- Supply Chain Management Integration:
- Seamless integration with supply chain management systems to correlate security events with cargo tracking and inventory data.
- Ensuring that cargo security monitoring aligns with overall supply chain visibility.
Training Required for Existing Staff:
- Operation of AI Surveillance Systems:
- Training security personnel on operating and interpreting results from AI-driven surveillance systems.
- Familiarization with the alerting and reporting system for efficient incident response.
- Interpreting Predictive Analytics:
- Education on understanding and acting upon predictions generated by the machine learning models.
- Training staff on leveraging predictive analytics for proactive security measures.
Challenges and Workarounds in Implementing the Tool:
- False Positives:
- Challenge: AI systems may generate false positives, leading to unnecessary alarms.
- Workaround: Implement a feedback loop to train the model and continuously reduce false positives over time.
- Privacy Concerns:
- Challenge: Deployment of surveillance systems raises privacy concerns among employees.
- Workaround: Implement transparent communication about security measures, ensuring compliance with privacy regulations.
Similar Tools already available in the market:
- Avigilon Control Center: Offers AI-powered video analytics for security and surveillance with features like facial recognition and object detection.
- BriefCam: Provides video content analytics for rapid review and search, enhancing security and operational efficiency.
Cost and Benefit Analysis:
- Costs:
- Development Costs:
- Investment in developing and fine-tuning AI algorithms and predictive analytics models.
- Costs associated with designing the alerting and reporting system.
- Hardware Costs: Expenses related to acquiring high-resolution cameras and powerful processing units.
- Development Costs:
- Benefits:
- Enhanced Security:
- Improved detection of security threats and rapid response capabilities.
- Reduction in security breaches and associated risks.
- Operational Efficiency:
- Streamlined incident response, leading to minimized disruptions in logistics operations.
- Enhanced visibility into security events for better decision-making.
- Enhanced Security:
Recommendation: Integrate with Similar Available Products
Considering the specialized nature of cargo security monitoring and the availability of advanced solutions, integration with AI Tools for Risk Management and Security in the Transportation and logistics industry, like Avigilon Control Center or BriefCam, is recommended.
Fraud Detection in Logistics
Business Knowledge Requirements:
- Logistics and Fraud Expertise:
- Understanding logistics operations and the specific fraud risks associated with transportation and supply chain activities.
- Familiarity with typical fraud schemes and techniques in the logistics industry.
- Transaction and Invoice Understanding:
- In-depth knowledge of transaction processes, invoicing systems, and financial interactions within the logistics domain.
- Understanding of legitimate transaction patterns to distinguish anomalies.
- Regulatory Compliance:
- Awareness of relevant regulations and compliance standards related to financial transactions in logistics.
- Knowledge of industry-specific fraud prevention guidelines and best practices.
Software Requirements:
- Machine Learning Algorithms:
- Development of machine learning models for pattern recognition to identify irregularities in transaction patterns, invoice data, and shipment routes.
- Implementation of anomaly detection algorithms for real-time monitoring of unusual behaviours.
- Real-time Monitoring Systems:
- Integration of real-time monitoring systems capable of processing and analyzing data streams to detect fraudulent activities as they occur.
- Deployment of algorithms that can adapt to changing patterns and behaviours over time.
- Data Integration and Analytics:
- Utilization of data integration tools to consolidate information from various sources such as transaction databases, shipment records, and invoice systems.
- Analytics capabilities to gain insights into historical fraud patterns and identify evolving threats.
Hardware Requirements:
- Processing Power:
- Adequate computing resources to support the processing demands of machine learning algorithms, especially for real-time analysis.
- Consideration of cloud-based solutions for scalability and flexibility.
Integration Requirements to Existing Toolset:
- Enterprise Resource Planning (ERP) Integration:
- Seamless integration with existing ERP systems to access transaction data, invoicing details, and financial information.
- Collaboration with financial management systems for a comprehensive view of financial transactions.
- Fraud Management Systems:
- Integration with existing fraud management tools to enhance the overall fraud detection capabilities.
- Collaboration with cybersecurity tools for a holistic approach to security.
Training Required for Existing Staff:
- Tool Usage Training:
- Training sessions to familiarize finance and logistics staff with the fraud detection tool, covering data input, system interpretation, and reporting procedures.
- Education on the importance of fraud prevention and the role of AI in enhancing security.
- Interpreting Anomalies:
- Training on interpreting anomalies detected by the system, distinguishing between false alarms and actual fraudulent activities.
- Ensuring staff can effectively collaborate with the AI system to investigate potential fraud cases.
Challenges and Workarounds in Implementing the Tool:
- False Positives:
- Challenge: The risk of generating false positives, leading to unnecessary investigations and disruptions.
- Workaround: Implement a feedback loop to continuously refine the algorithms, reducing false positives and improving accuracy.
- Data Quality and Integration:
- Challenge: Ensuring data quality and seamless integration with existing systems for a comprehensive view of transactions.
- Workaround: Implement data validation processes, address inconsistencies, and establish standardized data protocols.
Similar Tools already available in the market:
- SAS Fraud Management: Offers advanced analytics and machine learning capabilities for detecting and preventing fraud in various industries, including logistics.
- IBM Trusteer: Provides a comprehensive fraud detection solution with real-time capabilities and adaptive learning to combat evolving fraud techniques.
Cost and Benefit Analysis:
- Costs:
- Development Costs:
- Investment in developing and fine-tuning machine learning algorithms for pattern recognition and anomaly detection.
- Software development costs for real-time monitoring systems.
- Integration Costs:
- Resources required for seamless integration with existing ERP, fraud management, and cybersecurity systems.
- Potential customization costs for tailoring the tool to specific organizational needs.
- Training Costs:
- Budget for training finance and logistics staff on using the fraud detection tool and interpreting anomalies.
- Ongoing training to keep the staff updated on evolving fraud patterns.
- Development Costs:
- Benefits:
- Fraud Prevention:
- Mitigation of financial losses through early detection and prevention of fraudulent activities.
- Protection of the organization’s reputation by ensuring the integrity of financial transactions.
- Operational Efficiency:
- Streamlining fraud investigations and reducing manual efforts in monitoring transactions.
- Enhancing overall operational efficiency by preventing disruptions caused by fraud incidents.
- Fraud Prevention:
Recommendation: Integrate with Similar Available Products
Considering the complexity and specialized nature of fraud detection in logistics, integration with AI Tools for Risk Management and Security in the Transportation and logistics industry, such as SAS Fraud Management or IBM Trusteer, is recommended.
Real-Time Incident Response
Business Knowledge Requirements:
- Logistics Operations Understanding:
- In-depth knowledge of logistics and supply chain operations to comprehend the impact of security incidents on the overall flow of goods.
- Awareness of critical points in the logistics process where immediate response can mitigate risks.
- Security Protocols and Incident Response:
- Expertise in security protocols and incident response strategies specific to the transportation and logistics industry.
- Understanding of regulatory requirements related to incident reporting and response.
- IoT Integration Knowledge:
- Familiarity with IoT devices and sensors commonly used in logistics for tracking shipments, monitoring conditions, and gathering real-time data.
- Understanding of the potential data points and parameters crucial for incident response.
Software Requirements:
- AI-Driven Automation Systems:
- Development of AI-driven systems capable of automated response to security incidents, including decision-making algorithms for communication, rerouting, and protocol activation.
- Integration of natural language processing for effective communication with stakeholders.
- IoT Integration Software:
- Implementing software to connect and integrate with IoT devices and sensors enables real-time data exchange.
- Utilization of machine learning for data analysis and pattern recognition from IoT-generated data.
- Communication Platforms:
- Integration with communication platforms for instant alerts and notifications to relevant stakeholders.
- Secure and encrypted channels for communication to ensure data integrity and confidentiality.
Hardware Requirements:
- IoT Devices and Sensors:
- Deployment of various IoT devices and sensors, such as GPS trackers, temperature and humidity sensors, and security cameras, will be based on the specific requirements of the logistics operations.
- Adequate hardware infrastructure to support real-time data streaming from these devices.
- Processing Power: Sufficient computing power to handle the processing demands of AI-driven systems, especially during high-stakes incidents requiring rapid decision-making.
Integration Requirements to Existing Toolset:
- Supply Chain Management Integration:
- Seamless integration with supply chain management systems to access real-time data on shipments, inventory, and logistics operations.
- Collaboration with existing incident response tools to enhance overall capabilities.
- Communication Platform Integration:
- Integration with communication platforms the organization uses for streamlined and efficient stakeholder communication.
- Compatibility with existing notification systems to avoid disruptions.
Training Required for Existing Staff:
- Tool Familiarization:
- Training sessions to familiarize logistics and security personnel with the real-time incident response tool, covering its features, capabilities, and usage scenarios.
- Practice sessions for effective utilization during simulated incidents.
- IoT Device Management:
- Education on managing and interpreting data from various IoT devices and sensors integrated into the system.
- Training on identifying critical data points that trigger automated responses.
Challenges and Workarounds in Implementing the Tool:
- Data Accuracy and Reliability:
- Challenge: It is crucial to guarantee the accuracy and reliability of data from IoT devices to prevent false alarms or inadequate responses.
- Workaround: Implement data validation processes, conduct regular maintenance checks on IoT devices, and establish redundant systems for critical data points.
- Stakeholder Communication:
- Challenge: Efficiently communicating incidents to relevant stakeholders in real time.
- Workaround: Develop predefined communication templates, integrate with communication platforms, and conduct regular drills to optimize response times.
Similar Tools already available in the market:
- Everbridge: Provides incident response and crisis management solutions with real-time communication capabilities.
- Rave Mobile Safety: Offers emergency notification and incident management tools for immediate response to critical situations.
Cost and Benefit Analysis:
- Costs:
- Development Costs:
- Investment in developing sophisticated AI algorithms for automated incident response.
- Software development costs for IoT integration and communication platforms.
- Hardware Costs:
- Expenses related to acquiring and deploying a variety of IoT devices and sensors.
- Investment in computing infrastructure to support real-time data processing.
- Integration Costs:
- Resources required for seamless integration with existing supply chain management and communication systems.
- Potential customization costs for tailoring the tool to specific organizational needs.
- Development Costs:
- Benefits:
- Risk Mitigation:
- Immediate response to security incidents, minimizing the potential impact on logistics operations.
- Proactive measures to prevent the escalation of incidents and associated risks.
- Operational Efficiency:
- Streamlined incident response, reducing manual intervention and ensuring quicker resolution times.
- Enhanced overall logistics efficiency through automated decision-making.
- Reputation Protection:
- Protect the organization’s reputation by responding swiftly and effectively to security incidents.
- Increased trust from customers and stakeholders.
- Risk Mitigation:
Recommendation: Integrate with Similar Available Products
Integrating with established incident response, AI Tools for Risk Management and Security in the Transportation and Logistics industry, like Everbridge or Rave Mobile Safety, is recommended.
Biometric Authentication for Access
Business Knowledge Requirements:
- Logistics Security Protocols:
- Understanding of security protocols in the logistics industry, including access control requirements at critical points.
- Familiarity with regulatory compliance related to biometric authentication in sensitive areas.
- Biometric Technology Knowledge:
- In-depth knowledge of facial recognition, fingerprint scanning, and iris scanning technologies.
- Awareness of the strengths and limitations of each biometric modality in a logistics context.
- Access Control Policies:
- Expertise in defining and implementing access control policies specific to warehouses, loading docks, and transportation hubs.
- Understanding of the operational impact of restricting access to certain areas.
Software Requirements:
- Facial Recognition Algorithms:
- Development of facial recognition algorithms capable of accurately identifying individuals in various lighting and environmental conditions.
- Integration with machine learning for continuous improvement and adaptation to different faces.
- Fingerprint and Iris Scanning Software:
- Implementation of software for fingerprint and iris scanning, ensuring high accuracy and speed in the identification process.
- Integration with databases for storing and verifying biometric data.
- Access Control Software:
- Development of an access control software system that integrates biometric authentication with existing security infrastructure.
- User-friendly interfaces for managing access permissions and reviewing access logs.
Hardware Requirements:
- Biometric Scanners:
- Deployment of facial recognition cameras, fingerprint scanners, and iris scanners at critical access points.
- Ensuring compatibility and integration capabilities with the chosen software.
- Centralized Database:
- Establishment of a centralized database for storing and managing biometric data securely.
- Adequate storage capacity and redundancy measures for data integrity.
Integration Requirements to Existing Toolset:
- Security and Surveillance Integration:
- Seamless integration with existing security and surveillance systems to enhance overall security capabilities.
- Correlation of biometric access data with video footage for comprehensive access monitoring.
- Access Control Systems Integration:
- Integration with existing access control systems to synchronize biometric authentication with card-based or electronic access systems.
- Collaboration with security personnel for efficient monitoring.
Training Required for Existing Staff:
- Biometric System Usage Training:
- Training sessions for security personnel on using the biometric authentication system, including enrollment, troubleshooting, and data interpretation.
- Education on the importance of biometric security and its role in overall risk management.
- Access Control Policies:
- Training staff on new access control policies and procedures for implementing biometric authentication.
- Ensuring personnel understand the implications of restricted access areas.
Challenges and Workarounds in Implementing the Tool:
- Privacy Concerns:
- Challenge: Addressing privacy concerns related to collecting and storing biometric data.
- Workaround: Implement robust encryption measures, transparent communication with stakeholders, and adherence to privacy regulations.
- Environmental Factors:
- Challenge: Dealing with environmental factors affecting the accuracy of biometric identification, such as poor lighting conditions.
- Workaround: Implement additional sensors, such as infrared cameras, and regularly update algorithms for improved performance.
Similar Tools already available in the market:
- Suprema: Offers biometric access control solutions, including facial recognition, fingerprint scanning, and iris recognition.
- HID Global: Provides integrated biometric solutions for access control, including facial recognition and fingerprint technologies.
Cost and Benefit Analysis:
- Costs:
- Hardware Costs:
- Investment in biometric scanners, cameras, and other hardware components.
- Costs associated with setting up a centralized database and storage infrastructure.
- Software Development Costs:
- Development and refinement of facial recognition, fingerprint, and iris scanning algorithms.
- Investment in access control software development.
- Integration Costs:
- Resources required for seamless integration with existing security, surveillance, and access control systems.
- Customization costs for aligning the biometric authentication tool with specific logistics security requirements.
- Hardware Costs:
- Benefits:
- Enhanced Security:
- Strengthened access control measures with biometric authentication, reducing the risk of unauthorized access.
- Improved accuracy in identifying individuals compared to traditional access methods.
- Operational Efficiency:
- Streamlined access procedures, reducing the reliance on physical access cards or manual verification.
- Increased efficiency in monitoring and managing access permissions.
- Regulatory Compliance:
- Adherence to regulatory requirements related to secure access control in sensitive areas.
- Mitigation of legal risks associated with non-compliance.
- Enhanced Security:
Recommendation: Integrate with Similar Available Products
Integrating with established biometric access control, like Suprema or HID Global, is recommended.
AI-Enhanced Cybersecurity
Business Knowledge Requirements:
- Logistics Cybersecurity Understanding:
- In-depth knowledge of cybersecurity risks specific to the logistics industry, including potential threats to data integrity and supply chain operations.
- It would be advantageous to possess knowledge of the regulations and compliance standards pertinent to safeguarding data.
- Network Architecture Knowledge:
- Understanding the organization’s network architecture, including the logistics infrastructure’s interconnected systems and data flows.
- Knowledge of potential vulnerabilities in network design.
- Incident Response Expertise:
- Proficiency in incident response strategies, including containment, eradication, and recovery procedures for cybersecurity incidents.
- It is essential to comprehend the potential consequences of cyber threats on logistics operations.
Software Requirements:
- Predictive Threat Analysis Algorithms:
- Development of AI algorithms for predictive threat analysis, leveraging machine learning to identify potential cybersecurity threats based on network traffic patterns.
- Integration with threat intelligence feeds for real-time updates on emerging threats.
- Behavioural Analysis Systems:
- Implementation of AI-based systems for continuous monitoring of user and system behaviour.
- Using anomaly detection algorithms to identify unusual patterns that may indicate a cyber attack or unauthorized access.
- Security Information and Event Management (SIEM) Software:
- Integration with SIEM software for centralized logging, analysis, and correlation of security events across the logistics network.
- Collaboration with existing security infrastructure for comprehensive threat detection.
Hardware Requirements:
- Computing Power:
- Adequate computing resources to support the processing demands of AI algorithms for predictive threat analysis and behavioural analysis.
- Consideration of cloud-based solutions for scalability and flexibility.
Integration Requirements to Existing Toolset:
- SIEM Integration:
- Seamless integration with existing SIEM solutions to enhance overall cybersecurity capabilities.
- Correlation of AI-generated insights with data from other security tools for a comprehensive view of the threat landscape.
- Endpoint Protection Integration:
- Integration with endpoint protection systems to extend threat detection to individual devices within the logistics network.
- Collaboration with antivirus and anti-malware solutions for layered security.
Training Required for Existing Staff:
- Tool Usage Training:
- Training sessions for IT and cybersecurity personnel on using the AI-enhanced cybersecurity tool, covering configuration, monitoring, and response procedures.
- Hands-on exercises to simulate cyber threats and responses.
- Interpreting AI Insights: Education on interpreting insights generated by AI algorithms, understanding the context of threat alerts, and distinguishing between false positives and actual threats.
Challenges and Workarounds in Implementing the Tool:
- False Positives:
- Challenge: The risk of false positives, where the system flags normal behaviour as a potential threat.
- Workaround: Fine-tuning AI algorithms, incorporating user feedback, and continuously implementing a feedback loop to improve threat analysis accuracy.
- Data Privacy Concerns:
- Challenge: Balancing the need for enhanced cybersecurity with concerns about user privacy and data protection.
- Workaround: Implement anonymization measures, ensure compliance with data protection regulations, and transparent communication with stakeholders.
Similar Tools already available in the market:
- Darktrace: Offers AI-driven cybersecurity solutions, including predictive threat analysis and behavioural analysis for early threat detection.
- Vectra AI: Provides AI-powered cybersecurity solutions focused on detecting and responding to cyber threats using behavioural analysis.
Cost and Benefit Analysis:
- Costs:
- Development Costs:
- Investment in developing sophisticated AI algorithms for predictive threat analysis and behavioural analysis.
- Software development costs for integration with SIEM and other security tools.
- Integration Costs:
- Resources required for seamless integration with existing SIEM and endpoint protection systems.
- Potential customization costs for aligning the tool with specific logistics security requirements.
- Training Costs:
- Budget for training IT and cybersecurity staff on using the AI-enhanced cybersecurity tool and interpreting AI-generated insights.
- Ongoing training to keep the staff updated on evolving cyber threats.
- Development Costs:
- Benefits:
- Proactive Threat Detection: Early identification of potential cybersecurity threats through predictive analysis, allowing for proactive measures to prevent data breaches and system compromises.
- Behavioural Analysis: Continuous monitoring of user and system behaviour to identify unusual patterns indicative of cyber attacks or unauthorized access.
- Risk Mitigation: Mitigation of the impact of cybersecurity incidents on logistics operations through quick and informed incident response strategies.
Recommendation: Integrate with Similar Available Products
Integrating with established AI-driven cybersecurity solutions, like Darktrace or Vectra AI, is recommended.
Conclusion on AI tools for Risk Management and Security
In conclusion, developing AI tools for risk management and security in the logistics industry is a critical endeavour that demands a nuanced approach. The chosen tools, including Sustainable Sourcing Guidance, Cargo Security Monitoring, Fraud Detection in Logistics, Real-Time Incident Response, and Biometric Authentication for Access, reflect a comprehensive strategy to address diverse challenges in the industry.
For Sustainable Sourcing Guidance, the recommendation to integrate with existing solutions like Higg Index or EcoVadis is driven by the need for a swift and industry-tailored implementation. This approach aligns with the organization’s identity as an IT/AI pioneer, ensuring immediate deployment, cost efficiency, and access to established industry expertise.
Similarly, in Cargo Security Monitoring, Real-Time Incident Response, and Biometric Authentication for Access, integrating with proven tools such as Everbridge, Suprema, or Darktrace underscores the advantages of leveraging existing industry expertise, quick deployment, and cost-effectiveness. These solutions offer advanced features and mitigate the risks of building complex tools from scratch.
In AI-enhanced cybersecurity, integration with established solutions like Darktrace or Vectra AI is recommended for their proven track record in predictive threat and behavioural analysis. This choice aligns with the organization’s objective of efficient deployment, industry-specific expertise, and enhanced risk mitigation.
Ultimately, the integration approach ensures that the organization harnesses the benefits of cutting-edge AI tools while minimizing risks, staying agile in response to evolving industry needs, and maintaining a strategic focus on long-term viability and success in the dynamic transportation and logistics landscape.
Related Articles
- AI Tools for Predictive Analytics and Business Intelligence
- AI Tools for Sustainability and Green Logistics
- AI Tools for Compliance and Regulatory Support
- AI Tools for Fleet Maintenance and Monitoring
- AI Tools for Operational Efficiency and Automation
- AI Tools for Supply Chain Visibility and Collaboration
- AI Tools for Order and Shipment Tracking
- AI Tools for Warehouse Management and Automation
- AI Tools for Route Optimization and Planning
- AI tools in the Transportation and Logistics industry